前沿进展:在组合优化问题中重新审视采样
导语

目录
-
采样算法概述
-
高效的离散空间采样算法
-
采样算法与组合优化
-
采样算法与AI模型
1. 采样算法概述
1. 采样算法概述

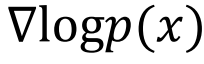
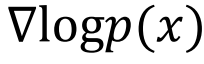
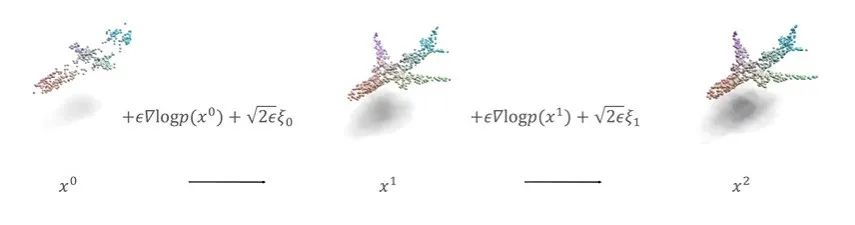
2. 高效的离散空间采样算法
2. 高效的离散空间采样算法
Sampling as first-order optimization over a space of probability measures [2022]. Anna Korba, Adil Salim. The variational formulation of the Fokker-Planck equation [1998]. Richard Jordan et al.
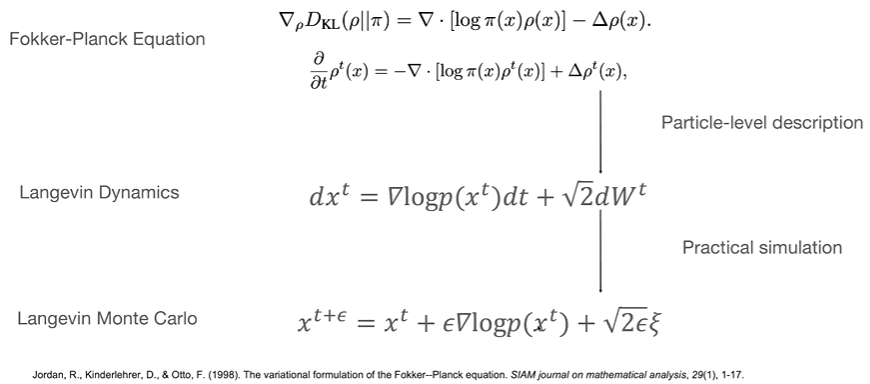

Fokker-Planck equations for a free energy functional or Markov process on a graph [2012]. Shui-Nee Chow et al.
Discrete Langevin sampler via Wasserstein gradient Flow [2023]. Haoran Sun et al. Thermodynamics of stoichiometric biochemical networks in living systems far from equilibrium [2005]. Hong Qian, Daniel A Beard. Path auxiliary proposal for MCMC in discrete space [2022]. Haoran Sun et al.
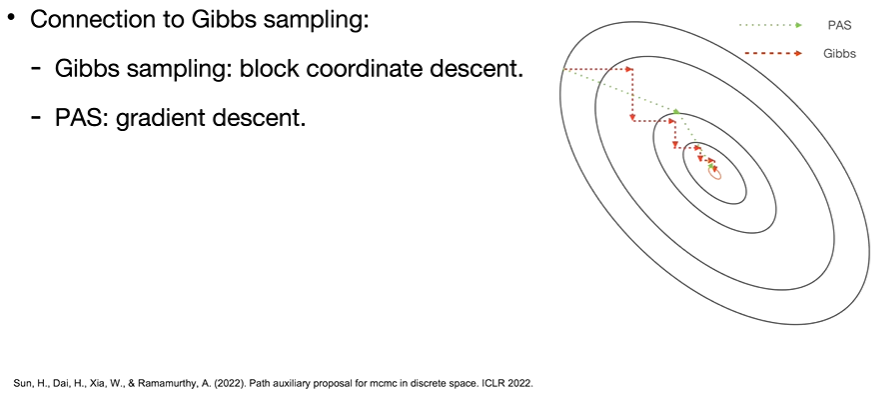
3. 采样算法与组合优化
3. 采样算法与组合优化


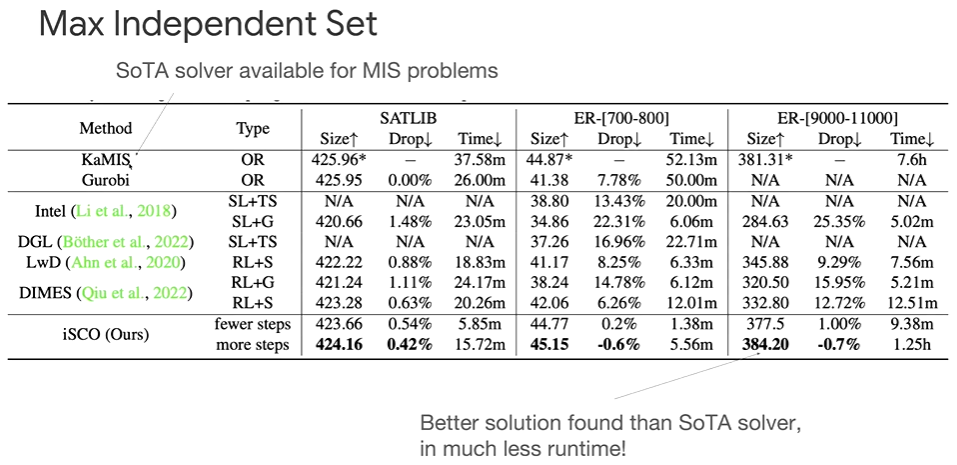
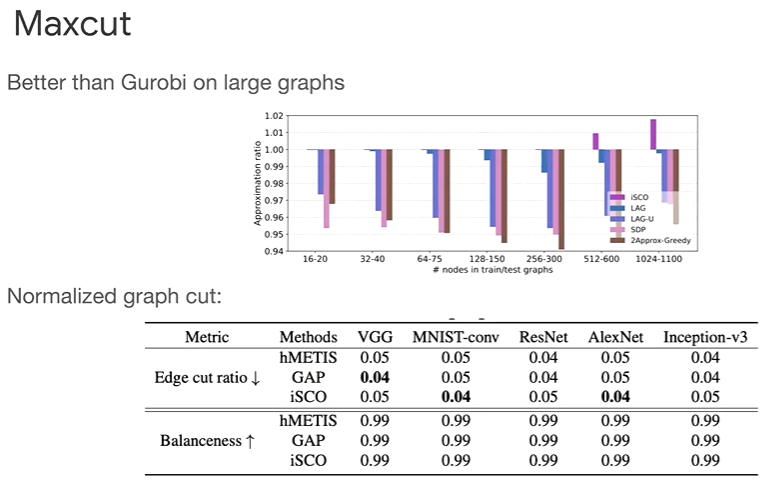
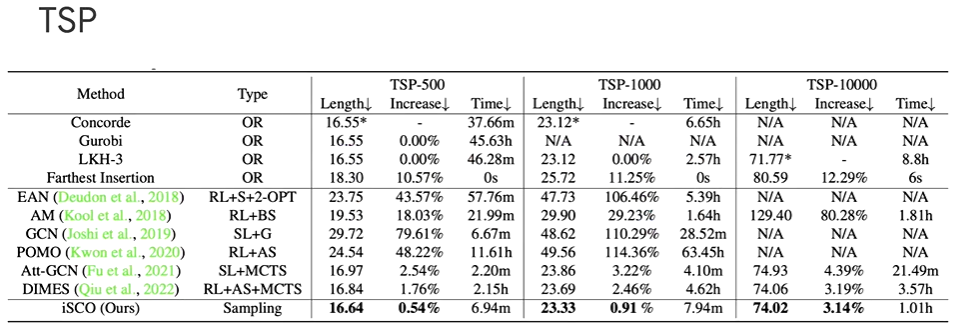
Revisiting Sampling for Combinatorial Optimization [2023]. Haoran Sun et al.
4. 采样算法与AI模型
4. 采样算法与AI模型
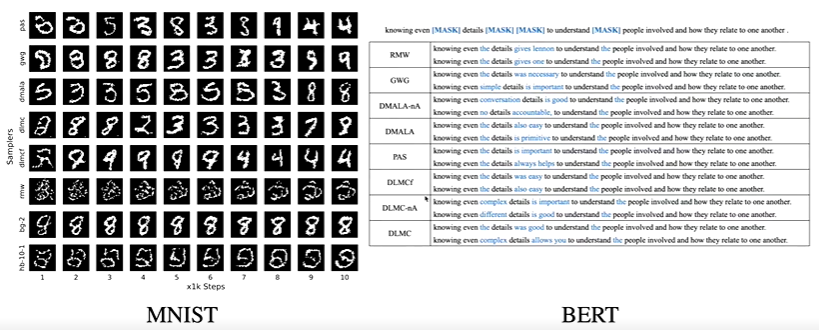
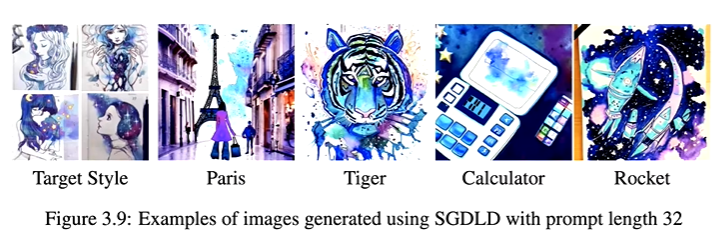
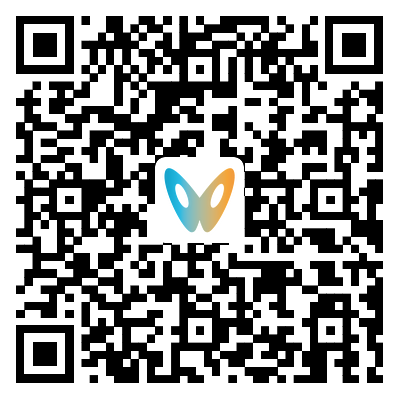
学者简介
戴涵俊,Google DeepMind实验室资深科学家和研究经理,此前于佐治亚理工获得博士学位。研究方向:高效的生成模型,包括大语言模型,图像和结构化数据模型,及其采样和优化的底层算法
图神经网络与组合优化读书会
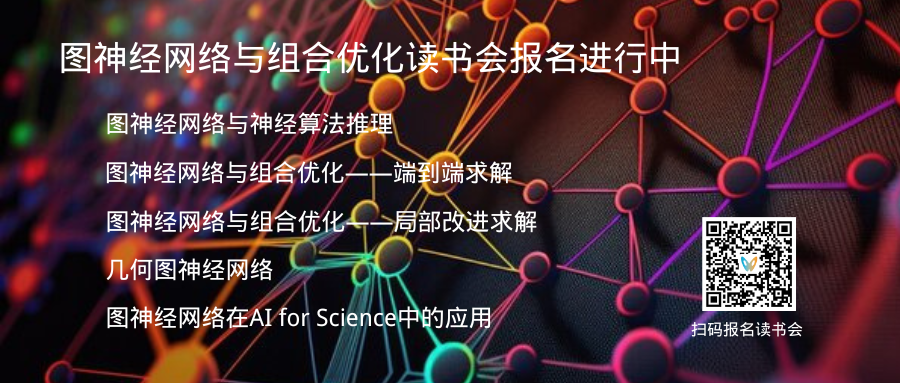
推荐阅读