PNAS速递:基于贝叶斯的人机混合预测框架
摘要
Artificial intelligence (AI) and machine learning models are being increasingly deployed in real-world applications. In many of these applications, there is strong motivation to develop hybrid systems in which humans and AI algorithms can work together, leveraging their complementary strengths and weaknesses. We develop a Bayesian framework for combining the predictions and different types of confidence scores from humans and machines. The framework allows us to investigate the factors that influence complementarity, where a hybrid combination of human and machine predictions leads to better performance than combinations of human or machine predictions alone. We apply this framework to a large-scale dataset where humans and a variety of convolutional neural networks perform the same challenging image classification task. We show empirically and theoretically that complementarity can be achieved even if the human and machine classifiers perform at different accuracy levels as long as these accuracy differences fall within a bound determined by the latent correlation between human and machine classifier confidence scores. In addition, we demonstrate that hybrid human–machine performance can be improved by differentiating between the errors that humans and machine classifiers make across different class labels. Finally, our results show that eliciting and including human confidence ratings improve hybrid performance in the Bayesian combination model. Our approach is applicable to a wide variety of classification problems involving human and machine algorithms.
人工智能和机器学习模型正越来越多地被应用于现实世界中。在许多这样的应用程序中,我们有很强的动机去开发人机混合系统。在人机混合系统中,人类和人工智能算法可以一起工作,充分利用它们具有的互补性优势和弱点。我们开发了一个贝叶斯框架,用于结合来自人类和机器的不同类型的预测的信心评分。该框架允许我们研究影响预测中的互补因素,在这种情况下,人机预测的混合组合结果比单独的人类预测及算法预测都显示出更好的表现。我们将这个框架应用到一个大规模数据集中,在这个数据集中,人类和各种卷积神经网络执行同样具有挑战性的图像分类任务。我们从经验和理论上证明,即使人机分类器呈现出不同的准确度水平上,只要人机分类器的准确度可信评分位于隐含的相关性所决定的范围内,也可以实现人机互补。此外,我们还证明了通过区分人类和机器分类器在不同类别标签之间产生的错误,可以提高混合人机系统的性能。最后,我们的结果表明,加入人给出的可信度评分可提高贝叶斯组合模型的混合性能。我们的方法适用于涉及人和机器算法的各种分类问题。
研究领域:预测模型,贝叶斯框架,人机混合系统

郭瑞东 | 作者
刘培源 | 审校
邓一雪 | 编辑
论文题目:
Bayesian modeling of human–AI complementarity 论文地址: https://www.pnas.org/doi/10.1073/pnas.2111547119
在预测任务中,使用算法得出的结果可能和人类判断不同,某些人类很难分辨的例子,对机器则很容易,反之亦有可能。最近发表在PNAS的一篇论文,尝试利用这样的互补性,来提升预测准确度。
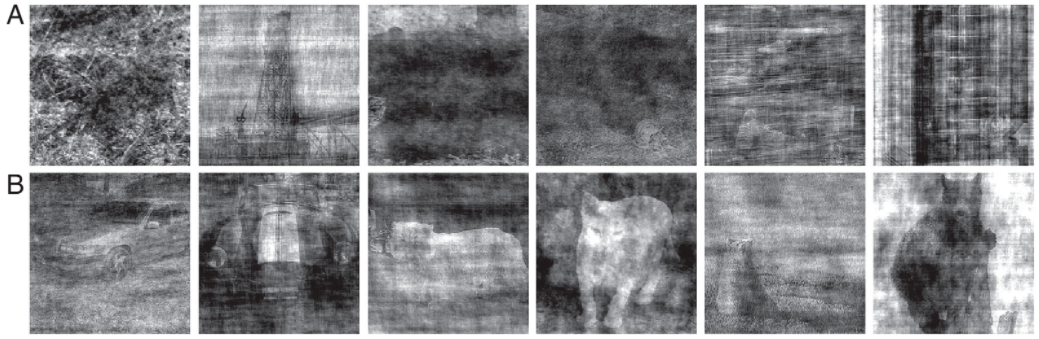
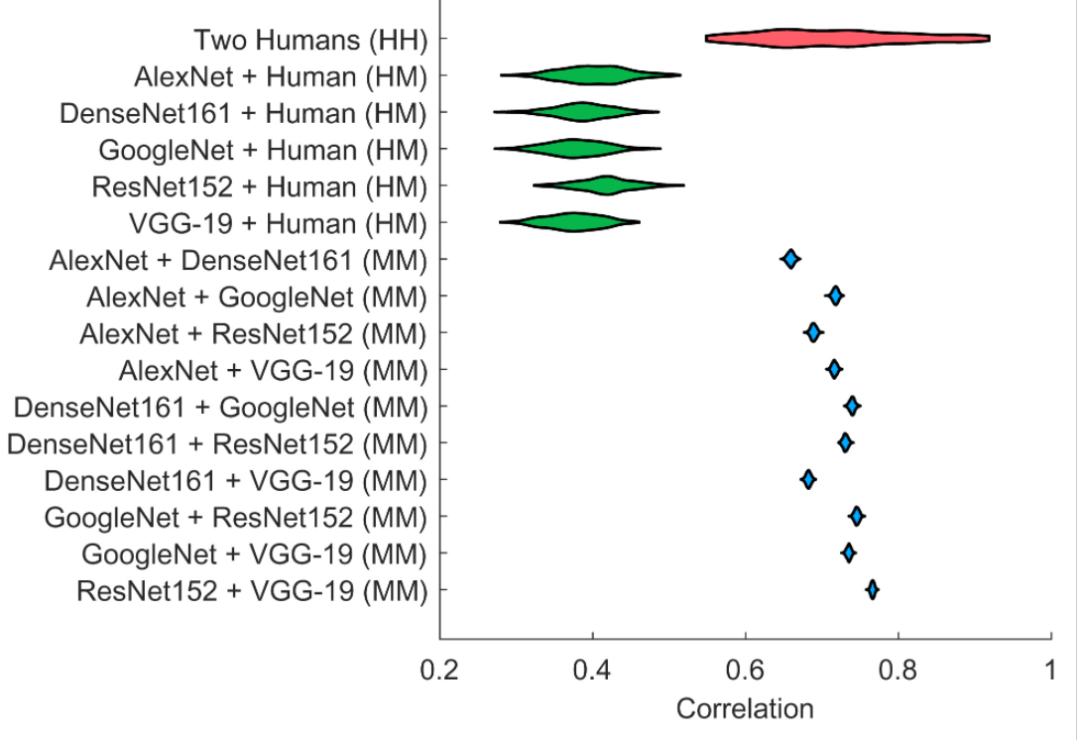
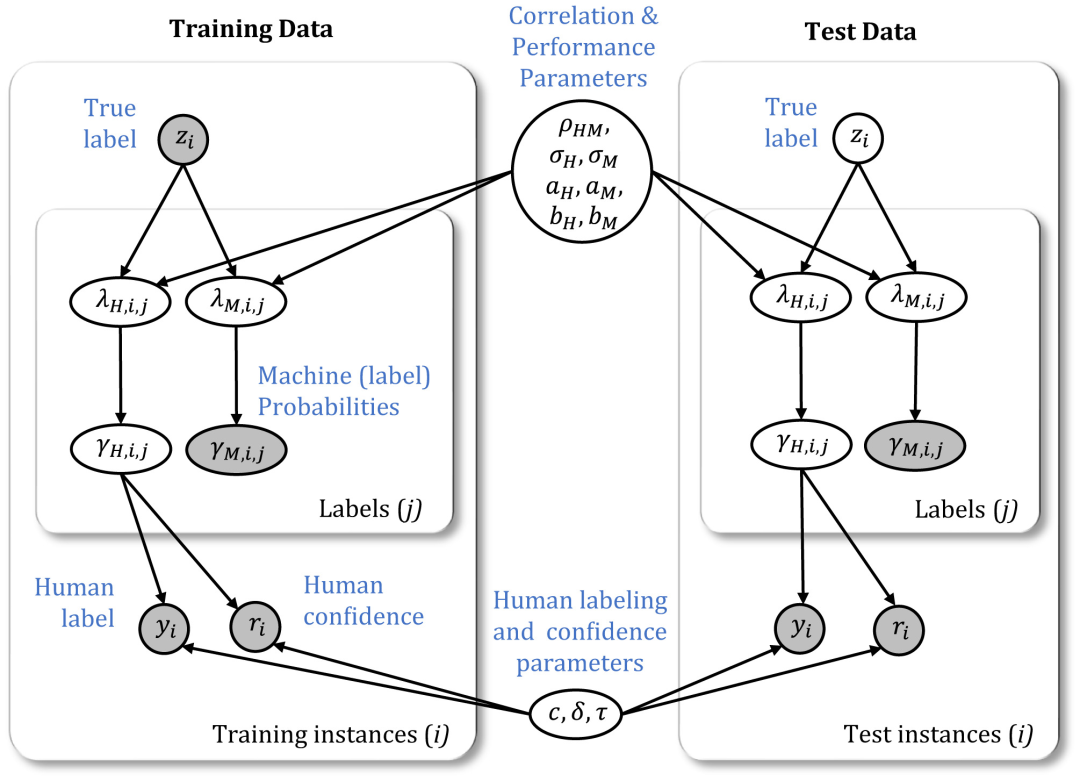
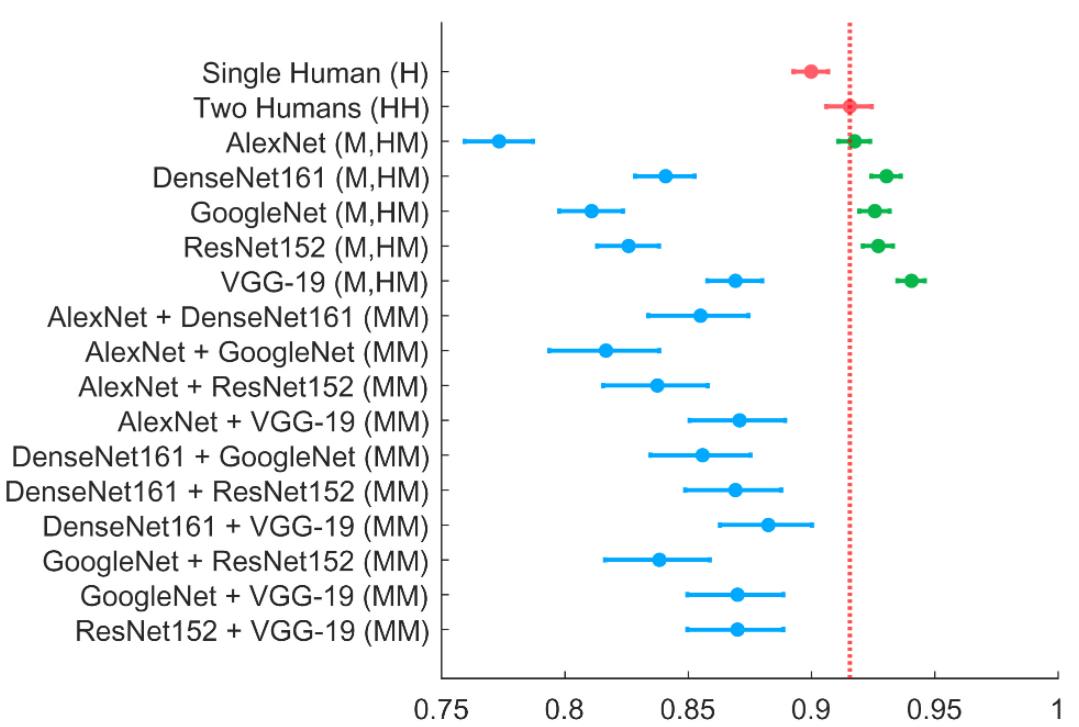
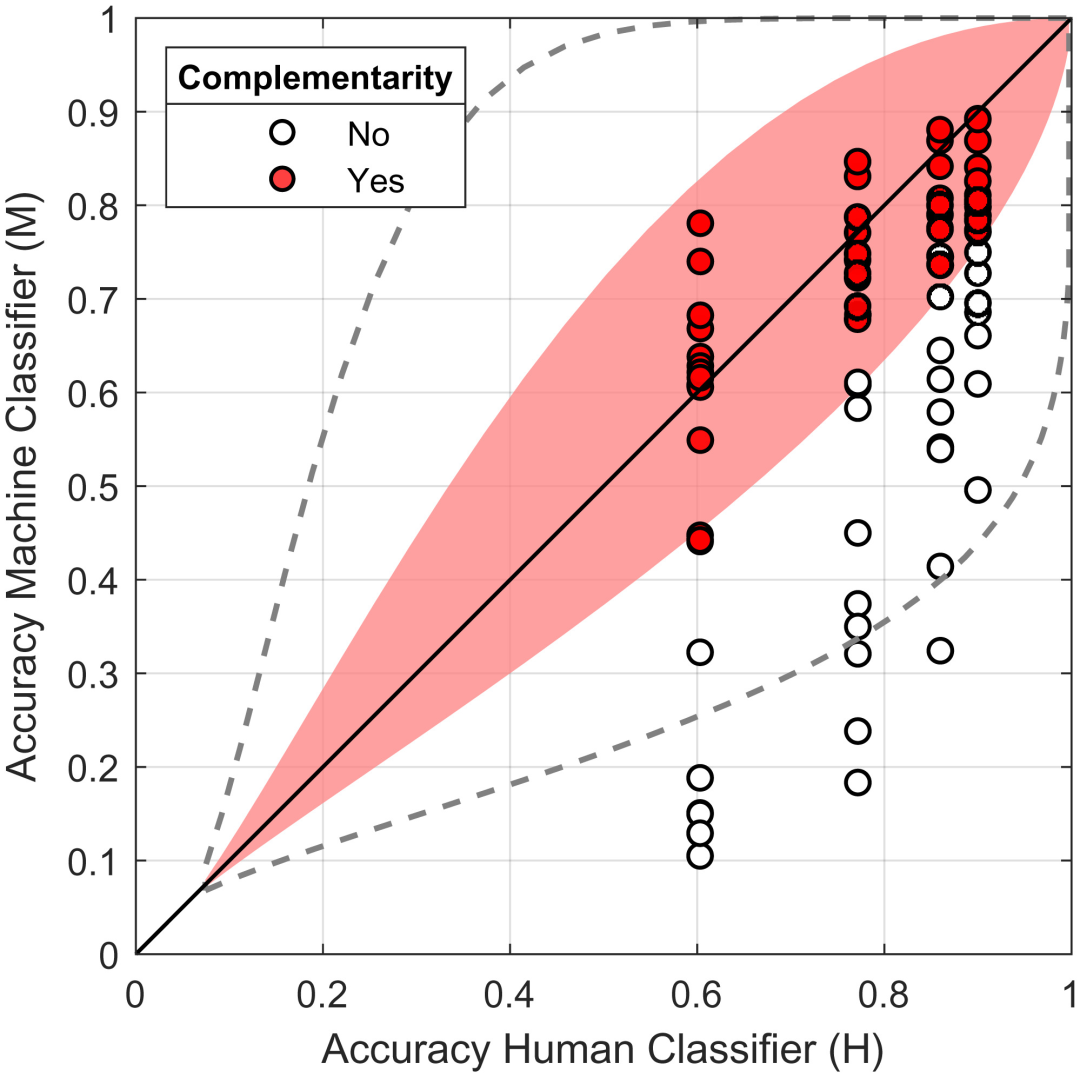
复杂科学最新论文
集智斑图顶刊论文速递栏目上线以来,持续收录来自Nature、Science等顶刊的最新论文,追踪复杂系统、网络科学、计算社会科学等领域的前沿进展。现在正式推出订阅功能,每周通过微信服务号「集智斑图」推送论文信息。扫描下方二维码即可一键订阅:
推荐阅读
点击“阅读原文”,追踪复杂科学顶刊论文