因果科学与 Causal AI 系列读书会 | 众包出书
集智俱乐部读书会是面向广大科研工作者的系列论文研读活动,其目的是共同深入学习探讨某个科学议题,了解前沿进展,激发科研灵感,促进科研合作,降低科研门槛。
读书会活动始于 2008 年,至今已经有 40 余个主题,内容涵盖复杂系统,人工智能,脑与意识,量子纠缠,张量网络,计算社会科学等。凝聚了众多优秀科研工作者,促进了科研合作发表论文,孵化了许多科研产品。如 2013 年的“深度学习”读书会孕育了彩云天气 APP,2015 年的“集体注意力流”读书会产生了众包书籍《走近2050》等。
背景
Judea Pearl 认为当下正在进行一场改变数据科学的新革命 — ”因果革命“。因果革命和以数据为中心的第一次数据科学革命,也就是大数据革命(涉及机器学习,深度学习及其应用,例如Alpha-Go、语音识别、机器翻译、自动驾驶等等 )的不同之处在于,它以科学为中心,涉及从数据到政策、可解释性、机制的泛化,再到一些社会科学中的归因和公平性问题,甚至哲学中的创造性和自由意志 。可以说, 因果革命彻底改变了科学家处理因果问题的方式,形成了一门新学科—因果科学。
当前的大多数 AI 系统只是曲线拟合,他们面临许多开放性难题本质上与因果相关,而其中的三个最主要困难 (1) 稳健性/适应性, (2) 可解释性,和 (3) 不能理解因果关系,而这些问题可以用现代因果建模解决。因果推理和机器学习融合,构建具备果推理能力的 Causal AI 系统,是实现强人工智能的关键步骤。
To Build Truly Intelligent Machines, Teach Them Cause and Effect
— Judea Pearl
为此,我们希望可以通过因果推理的专题读书会,来梳理因果科学的核心内容,理解它如何改变数据科学,助力 AI 系统超越曲线拟合和获得回答因果问题的能力。
读书会目的
读书会目的
参与方式及时间
参与人员:
运行模式:
具体规则:
-
贡献了一次讲座(半小时以上)内容的(需要提前向主持人申请并通过试讲);
-
完成了一篇以上读书笔记写作的(读书笔记标准:字数3千以上,图文并茂,具体请参照此文:因果观念新革命?万字长文,解读复杂系统背后的暗因果);
-
复现读书会中某篇讲读论文的结果并提交代码;
-
由读书会内容启发,产生了靠谱的新产品创意,并在读书会结束 2 个月内提交了详细的产品策划方案,并通过了集智俱乐部组织的相应考核答辩的;
-
由读书会内容启发,萌发了科研论文创意,并在读书会结束 2 个月内完成初稿,并通过了集智俱乐部组织的相应考核答辩的;
报名:(长期有效)
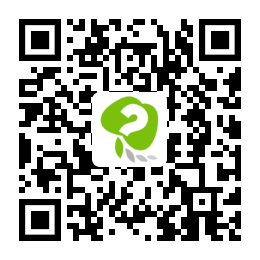
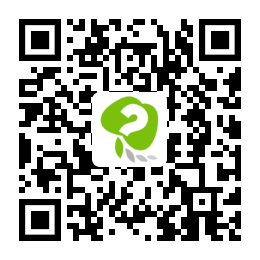
第一步:扫码填写报名信息。
第二步:信息填写之后,会弹出对应的课程购买信息,提交保证金299元。(符合退费条件后可退费)。
第三步:添加负责人微信,拉入对应的读书会讨论群。
论文清单
读书会会分享如下经典教材中有关因果推理基础内容
深刻理解因果之梯
-
Bareinboim, E., et al. “On Pearl’s hierarchy and the foundations of causal inference.” ACM Special Volume in Honor of Judea Pearl (provisional title) (2020).
简介:Judea Pearl 的学生 Elias Eareinboim 亲自介绍了 Pearl 的因果之梯
机器学习+因果推理综述
-
Schölkopf, Bernhard. “Causality for machine learning.” arXiv preprint arXiv:1911.10500 (2019).
简介:这是一篇刚刚挂在arxiv 就被 Pearl 亲自 twitter 点赞的论文,是马普智能所所长 Bernhard Schölkopf 最引以为傲的论文之一,他把被 Pearl 点赞这事情第一时间写在个人主页自我介绍的第一段中。Schölkopf 及其团队在因果结合机器学习方面做了最多的工作,此文总结并升华了信息革命时代下因果结合机器学习的一般理论和深刻思考
-
Malinsky Daniel, Ilya Shpitser, and Thomas S. Richardson. “A Potential Outcomes Calculus for Identifying Conditional Path-Specific Effects.” international conference on artificial intelligence and statistics (2019): 3080-3088.
简介:当前有两个主流因果建模框架,潜结果(Potential Outcome)和结构因果模型(SCM),它们结合起来产生了 Po-calculus
机器学习方法+因果推理
-
Kuang, Kun, et al. “Stable prediction across unknown environments.” Proceedings of the 24th ACM SIGKDD International Conference on Knowledge Discovery & Data Mining. 2018.
-
Shen, Zheyan, et al. “Stable Learning via Sample Reweighting.” national conference on artificial intelligence (2020).
-
Kuang, Kun, et al. “Stable Prediction with Model Misspecification and Agnostic Distribution Shift.” national conference on artificial intelligence (2020).
-
Sgouritsa, Eleni, et al. “Inference of Cause and Effect with Unsupervised Inverse Regression.” international conference on artificial intelligence and statistics (2015): 847-855.
简介:半监督学习理论上只对反因果问题有效
-
Wager, Stefan, and Susan Athey. “Estimation and Inference of Heterogeneous Treatment Effects using Random Forests.” Journal of the American Statistical Association 113.523 (2018): 1228-1242.
简介:用因果随机森林的办法来学习异质因果效应
Niklas Pfister, P. Bühlmann, J. Peters 团队 Invariant causal prediction 系列文章
-
Peters, Jonas, Peter Buhlmann, and Nicolai Meinshausen. “Causal inference using invariant prediction: identification and confidence intervals.” arXiv: Methodology (2015).
-
Pfister, Niklas, Peter Buhlmann, and Jonas Peters. “Invariant Causal Prediction for Sequential Data.” Journal of the American Statistical Association 114.527 (2019): 1264-1276.
-
Arjovsky, Martin, et al. “Invariant Risk Minimization.” arXiv: Machine Learning (2019).
简介:对于不同数据和模型,学习具备 Out-of-distribution 泛化能力的预测模型
强化学习+因果推理
-
Zhu, Shengyu, Ignavier Ng, and Zhitang Chen. “Causal Discovery with Reinforcement Learning..” arXiv: Learning (2019).
简介:使用强化学习来做因果发现
-
Zhang, Junzhe, Daniel Kumor, and Elias Bareinboim. “Causal Imitation Learning with Unobserved Confounders.” (2020).
简介:有未观测混杂因子下的因果模仿学习
-
Pearl, Judea, and Elias Bareinboim. “Transportability of causal and statistical relations: a formal approach.” national conference on artificial intelligence (2011): 247-254.
简介:半监督学习理论上只对反因果问题有效
-
Correa, Juan D., and Elias Bareinboim. “General Transportability of Soft Interventions: Completeness Results.” (2020).
简介:因果可迁移行理论的一般性框架
中介分析理论
-
Robins, James M., Thomas S. Richardson, and Ilya Shpitser. “An interventionist approach to mediation analysis.” arXiv preprint arXiv:2008.06019 (2020).
简介:半监督学习理论上只对反因果问题有效
-
Miles, Caleb H., et al. “On semiparametric estimation of a path-specific effect in the presence of mediator-outcome confounding.” Biometrika 107.1 (2020): 159-172.
简介:中介分析在社会学科中有重要应用,涉及到公平性,归因等等问题
因果推理+医疗大数据
-
Prosperi, Mattia, et al. “Causal inference and counterfactual prediction in machine learning for actionable healthcare.” Nature Machine Intelligence 2.7 (2020): 369-375.
简介:通过反事实推断,制定健康干预方案,相比基于相关性的方案,提升对用户的准确性
-
Richens, Jonathan G., Ciarán M. Lee, and Saurabh Johri. “Improving the accuracy of medical diagnosis with causal machine learning.” Nature Communications 11.1 (2020): 1-9.
简介:用包含因果推理的机器学习模型,提升医疗诊断的准确性
-
Castro, Daniel C., Ian Walker, and Ben Glocker. “Causality matters in medical imaging.” Nature Communications 11.1 (2020): 1-10.
简介:医学影像中,通过引入因果推理,提升可解释性和稳健性
-
Saxe, Glenn N., et al. “Computational causal discovery for post-traumatic stress in police officers.” Translational psychiatry 10.1 (2020): 1-12.
简介:通过因果发现,基于观察数据,找出心理疾病的成因
时间序列+因果推理
-
Runge, Jakob, et al. “Inferring causation from time series in Earth system sciences.” Nature communications 10.1 (2019): 1-13. 简介:专注地球大气系统时间序列的因果推理方法学 -
Runge, Jakob, et al. “Detecting and quantifying causal associations in large nonlinear time series datasets.” Science Advances 5.11 (2019): eaau4996.
简介:大数据集下的因果发现及定量方法
-
Reid, Andrew T., et al. “Advancing functional connectivity research from association to causation.” Nature neuroscience 22.11 (2019): 1751-1760.
简介:在针对大脑功能的研究中,如何从相关关系中找到可能的因果联系
-
Marinescu, Ioana E., Patrick N. Lawlor, and Konrad P. Kording. “Quasi-experimental causality in neuroscience and behavioural research.” Nature human behaviour 2.12 (2018): 891-898.
简介:借鉴计量经济学的方法,从神经科学及认知科学中找到因果关系
-
Varian, Hal R. “Causal inference in economics and marketing.” Proceedings of the National Academy of Sciences 113.27 (2016): 7310-7315.
简介:因果推理在经济学和营销中的应用
-
Xie, Yu. “Population heterogeneity and causal inference.” Proceedings of the National Academy of Sciences 110.16 (2013): 6262-6268.
简介:在包含异质人口的数据中,找到因果关系
复杂系统+因果推理
-
Xie, Yu. “Population heterogeneity and causal inference.” Proceedings of the National Academy of Sciences 110.16 (2013): 6262-6268.
简介:在包含相互作用的因果链条的系统中,拆解因果链条
-
Leng, Siyang, et al. “Partial cross mapping eliminates indirect causal influences.” Nature communications 11.1 (2020): 1-9.
简介:通过交叉映射,找到直接的因果关系
-
Weissman, Michael B. “Do GRE scores help predict getting a physics Ph. D.? A comment on a paper by Miller et al.” Science Advances 6.23 (2020): eaax3787.
简介:通过因果推理,反驳之前的教育学研究的方法学缺陷,指出其结论有误
-
Ferraro, Paul J., James N. Sanchirico, and Martin D. Smith. “Causal inference in coupled human and natural systems.” Proceedings of the National Academy of Sciences 116.12 (2019): 5311-5318.
简介:在人类及自然环境相互影响的环境中,进行因果推理
关于众包生产书籍
集智俱乐部自2015年以来,充分调动社区成员的积极性,组织以众包的方式,创作和翻译4本图书作品,分别是:

此次,我们依然希望通过读书会+众包的形式可以完成《因果推理在数据科学中的应用》一书的生产与撰写,希望感兴趣的朋友们快来加入我们!
初版大纲:
推荐阅读
集智俱乐部QQ群|877391004
商务合作及投稿转载|swarma@swarma.org
◆ ◆ ◆
搜索公众号:集智俱乐部
加入“没有围墙的研究所”
让苹果砸得更猛烈些吧