因果科学算法、框架、数据集汇总
导语
本文收集了相关的概率编程框架、工具包、数据集及基准,并依此进行分类。特别感谢因果社区成员闫和东的梳理和总结,感谢龚鹤扬、张天健、李奉治、段月然、孙钦贵参与讨论和贡献,我们后续会对相应的算法做更详细的介绍和说明,请对相关内容感兴趣的同学或者老师加入因果社区,一起贡献!

1. 简介
1. 简介
因果科学的工作大致可以分为基础因果假设及框架(fundamental causal assumption and framework)、因果学习(causal learning)、因果推断(causal reasoning/inference)和应用系统,其中因果学习又可以分为因果结构学习(causal discovery/causal structure learning)和因果表示学习(causal representation learning)。
本文收集了相关的概率编程框架、工具包、数据集及基准,并依此进行分类。
2. 概率编程框架
2. 概率编程框架
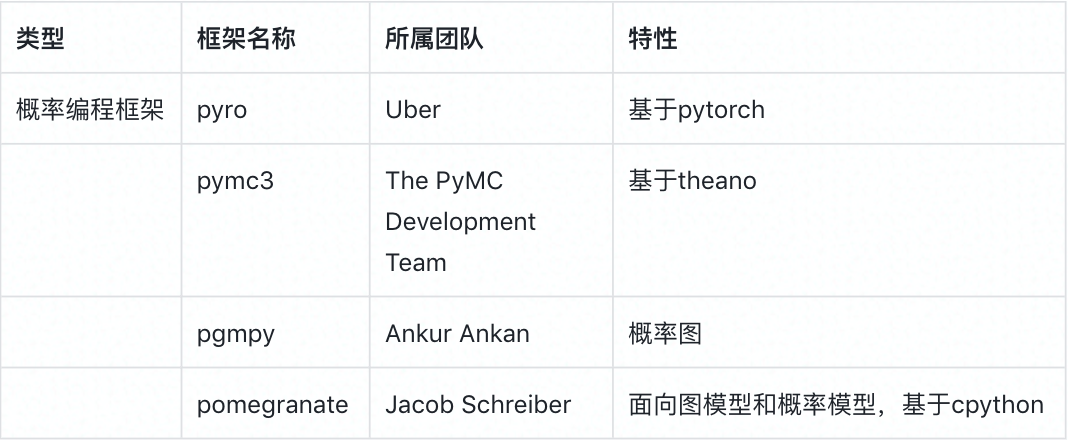
相关链接:
pyro:
pymc3:
pgmpy:
pomegranate:
https://github.com/jmschrei/pomegranate
3. 工具包
3. 工具包
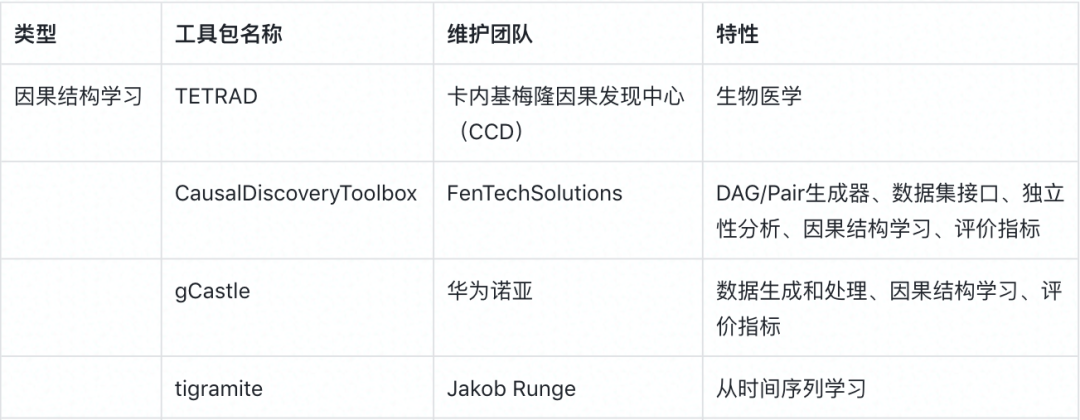

相关链接:
TETRAD:
CausalDiscoveryToolbox:
https://github.com/FenTechSolutions/CausalDiscoveryToolbox
gCastle:
tigramite:
Ananke:
EconML:
dowhy:
causalml:
WhyNot:
CausalImpact:
Causal-Curve:
grf:
dosearch:
causalnex:
https://github.com/quantumblacklabs/causalnex
4. 数据集或基准
4. 数据集或基准
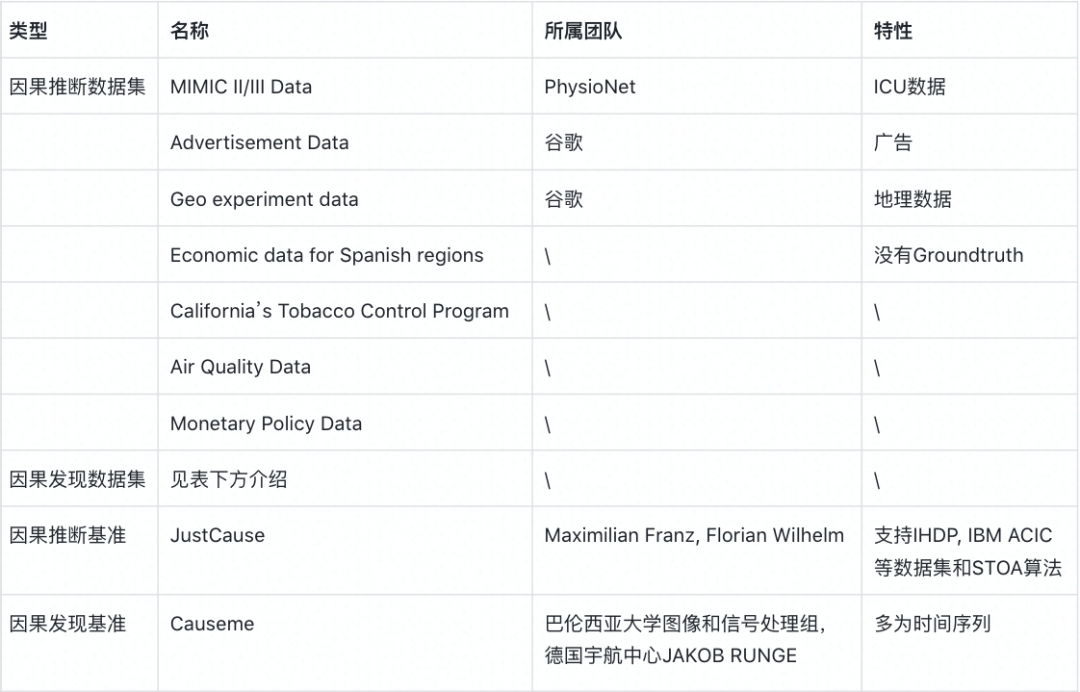
相关链接:
MIMIC II/III Data:
https://archive.physionet.org/mimic2/
Advertisement Data:
Geo experiment data:
Economic data for Spanish regions:
California’s Tobacco Control Program:
Air Quality Data:
Monetary Policy Data:
JustCause:
Causeme:
因果发现数据集
参考综述文献:https://arxiv.org/abs/2102.05829
真实数据集:US Manufacturing Growth Data,Diabetes Dataset,Temperature Ozone Data,OHDNOAA Dataset ,Neural activity Dataset,Human Motion Capture,Traffic Prediction Dataset,Stock Indices Data
合成数据集:Confounding/ Common-cause Models,Non-Linear Models,Dynamic Models, Chaotic Models
5. 其他相关链接或参考
5. 其他相关链接或参考
郭若城:
https://github.com/rguo12/awesome-causality-algorithms
欢迎加入因果科学社区
欢迎加入因果科学社区
因果推断与机器学习领域的结合已经吸引了越来越多来自学界业界的关注,为深入探讨、普及推广因果科学议题,智源社区携手集智俱乐部连续举办了两季「因果科学与CausalAI读书会」,研读重要论文与经典教材。
目前因果科学读书会(即因果科学社区),已经有超过600位海内外相关科研工作者以及互联网一线从业人员参与,如果你也对这个主题感兴趣,想要深度地参与,就快加入我们吧!
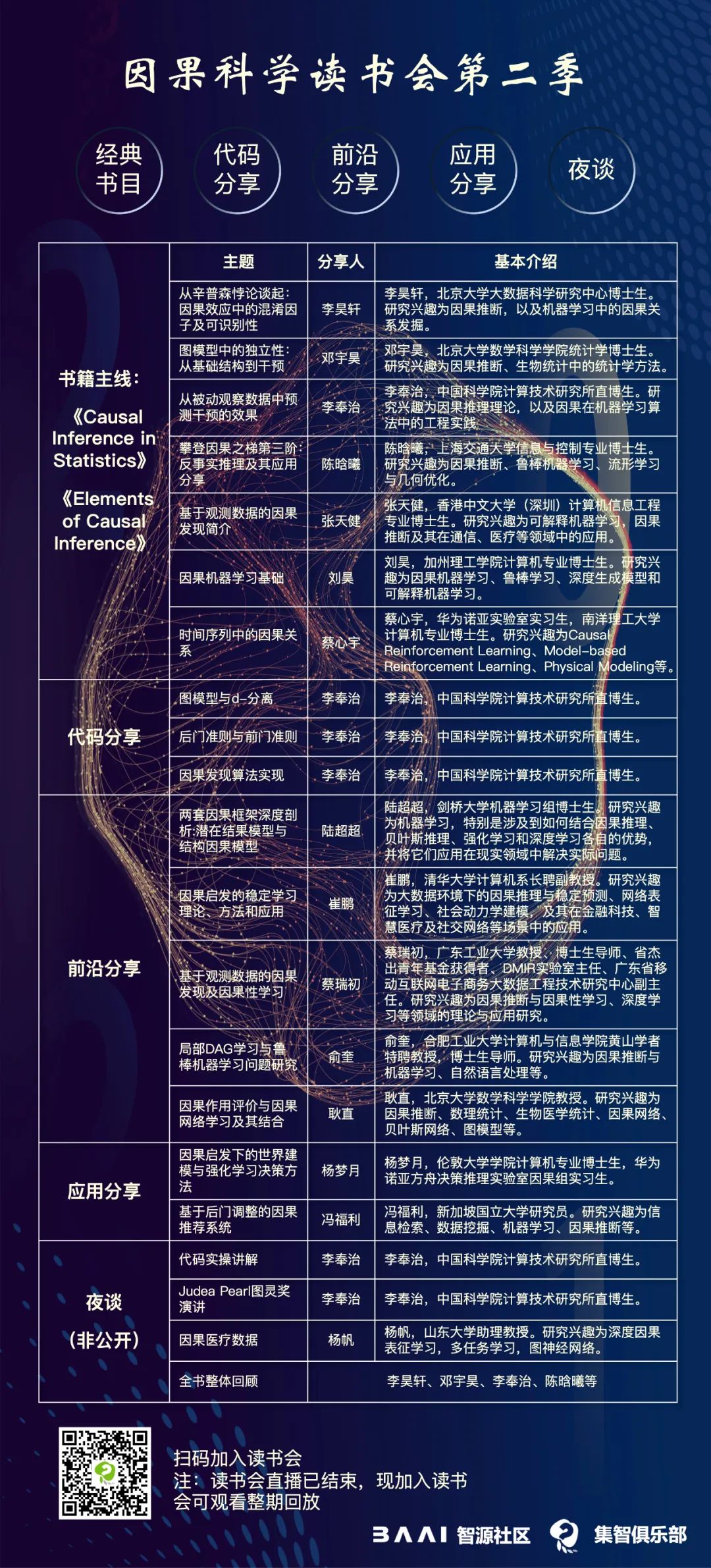
推荐阅读
点击“阅读原文”,即可报名读书会