第二届神经网络新年交叉论坛
2022-12-27
1,512
深度神经网络正在成为AI for Science的基本工具,构成新的科学研究范式,以前所未有的广度和深度影响科学的发展。破解深度网络的机制使得人们能从第一性原理出发设计更好的网络结构和算法,甚至有助于理解生物智能的本质。神经网络新年交叉论坛于2021年底由一群热衷于交叉学科研究的青年科学家创立,邀请来自不同学科背景的科学家进行人工(生物)智能原理方面的年度研讨。
第二届神经网络新年交叉论坛将于2022年12月31~2023年1月1日举行(线上)。该论坛已成功举办了第一届 (蔲享链接:https://www.koushare.com/lives/room/506610) 。第二届将邀请在人工智能,数学物理和脑科学交叉领域从事基础研究的科学家分享领域前沿和未来趋势展望。
论坛学术委员:毕则栋、陈国璋、黄海平、雷泽、黎勃、库逸轩、孟祥明、魏宪,余肇飞、杨冬平,张文昊、张希昀
蔻享直播 (可扫码观看):
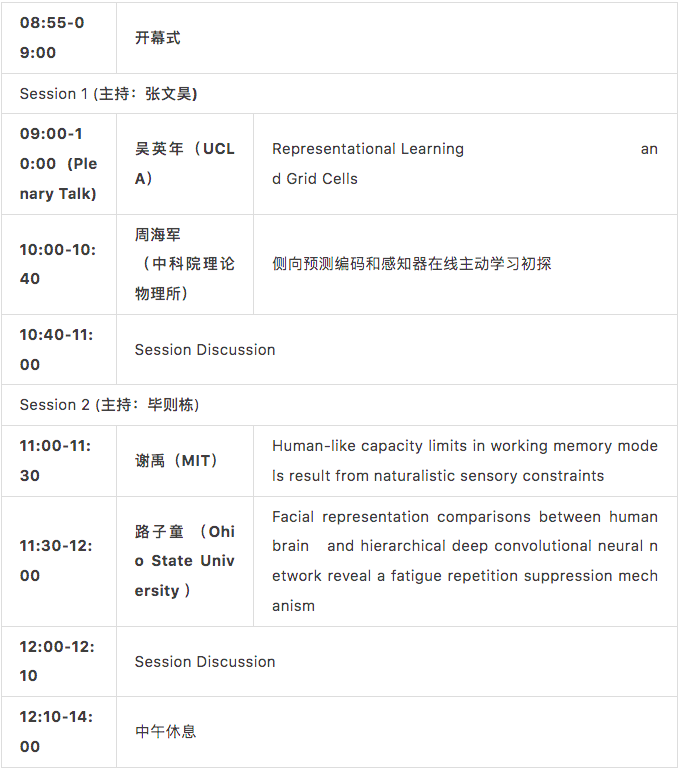
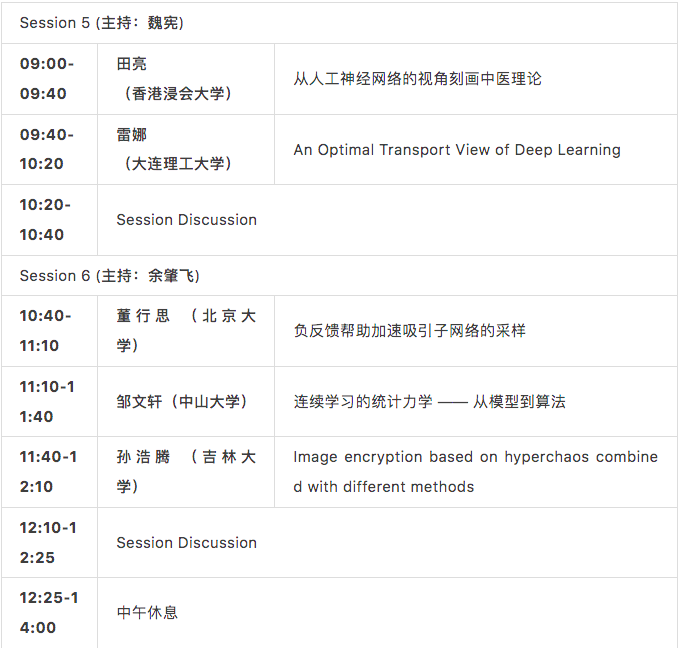
Representational Learning and Grid Cells
A key perspective of deep learning is representation learning, where concepts or entities are embedded in latent spaces and are represented by latent vectors whose elements can be interpreted as activities of neurons. In this talk, I will discuss our recent work on representational models of grid cells. The grid cells in the mammalian entorhinal cortex exhibit striking hexagon firing patterns when the agent (e.g., a rat or a human) navigates in the 2D open field. I will explain that the grid cells collectively form a vector representation of the 2D self-position, and the 2D self-motion is represented by the transformation of the vector. We identify a group representation condition and a conformal isometry condition for the transformation, and show that these two conditions lead to locally conformal embedding and the hexagon grid patterns. Joint work with Ruiqi Gao, Jianwen Xie, Wenhao Zhang, Xue-Xin Wei and Song-Chun Zhu.
近年来,神经网络的统计物理物理学越来越引起人们的关注。从自旋玻璃和组合优化的角度看待神经网络的参数学习问题是一个重要视角。神经网络的学习过程,可以看成是在网络中建立一个内部模型表征外部世界的过程。我将汇报我们在侧向预测编码和感知器在线主动学习方面的一点点理论和实证结果,重点在侧向预测编码。对于侧向预测编码问题,我也将展示最优预测权重矩阵的对称性发生改变的一些初步数值计算结果。
Human-like capacity limits in working memory models result from naturalistic sensory constraints
Working memory (WM) allows us to hold information temporarily and make complex decisions beyond reflexive response to stimuli. One prominent feature of WM is its capacity limit. Despite decades of study, the root of this limit is still not well-understood. Most previous accounts for this limit assume various forms of memory constraints and make strong, often oversimplified, assumptions about sensory representations of stimuli. In this work, we built visual-cognitive neural network models of WM that process raw sensory stimuli. In contrast to intuitions that capacity limit results from memory constraints, we found that pre-training the sensory region of our models with natural images poses enough constraints on models to exhibit human-like behavior patterns across a wide range of WM capacity tasks. In change detection tasks, the detection accuracy decreases rapidly when the number of stimuli to be remembered increases. In continuous report tasks, the fidelity of the report again decreases rapidly when more stimuli are shown. In contrast, models without realistic constraints on the sensory regions produce super-human performance in these tasks. Human-like behavior cannot be restored simply by restricting the size of these models or adding processing noise. Unlike phenomenological accounts of WM capacity, our neural network models allow us to test the neural mechanisms of capacity limitation. We found that the average neural activation in our model increases and then plateaus when more stimuli are presented, and capacity limitation appears to arise in a bottom-up fashion; both are broadly consistent with previous fMRI and electrophysiological studies. Our work suggests that many phenomena about WM capacity can be explained by sensory constraints. Our models offer a fresh perspective in our understanding of the origin of the WM capacity limit and highlight the importance of building models with realistic sensory processing even when studying memory and other high-level cognitive phenomena.
路子童 (Ohio State University )
Facial representation comparisons between human brain and hierarchical deep convolutional neural network reveal a fatigue repetition suppression mechanism.
Repetition suppression (RS) for faces, a phenomenon that neural responses are reduced to repeated faces in the visual cortex, have long been studied. However, the underlying primary neural mechanisms of RS remains debated. In recent years, artificial neural networks can achieve the performance of face recognition at human level. In our current study, we combined human electroencephalogram (EEG) and a hierarchical deep convolutional neural network (DCNN) and applied reverse engineering to provide a novel way to investigate the neural mechanisms of facial RS. First, we used brain decoding approach to explore the representations of faces and demonstrates its repetition suppression effect in human brains. Then not only we investigated how facial information was encoded in DCNN, but also we used DCNN as a tool to simulate the neural machanism of facial repetition suppression as fatigue or sharpening of neurons and compared representations between human brains and DCNNs. In fatigue hypothesis, we assumed that the activation of neurons with stronger response to face stimulus would be attenuated under the repetition condition. In sharpening hypothesis, we assumed that the neurons with weak response to face stimulus would be not activated any more under the repetition condition. As a core part, we constructed two RS models, fatigue and sharpening models, to modify the activations of DCNNs and conducted cross-modal representational similarity analysis (RSA) comparisons between dynamic processing in human EEG signals and layers in modified DCNNs respectively. We found that representations of human brains were more similar to those in fatigue-modified DCNN, compared with sharpening modified DCNN. Also, human brains showed stronger and longer similarities with the fatigue-modified DCNN as the layer increased. Our results suggests that the facial RS effect in face perception is more likely caused by the fatigue mechanism suggesting that the activation of neurons with stronger responses to face stimulus would be attenuated more. Therefore, the current study supports the fatigue mechanism as a more plausible neural mechanism of facial RS. The comparison between representations in the human brain and hierarchical DCNN provides a promising tool to simulate and infer the brain mechanism underlying human behaviors.
摘要:本报告首先简要概述了下一代神经质量模型,它代表了异构脉冲神经网络精确平均场模型发展的新视角。然后我将报告应用这种方法来重现神经科学中观察到的相关实验现象的结果,范围从慢速和快速伽马振荡到兴奋性神经元驱动的类伽马振荡以及theta振荡嵌套的伽马振荡。最后,我将展示这些神经质量模型如何扩展以捕获由无序的动力源引起的扰动驱动现象,这些扰动自然存在于大脑回路中,例如背景噪声或者由于神经元之间的稀疏连接而导致的电流扰动。
Classically, neurogenesis (birth of neurons) was impossible for adult mammals. Since the second half of the last century, evidence has suggested that adult-born neurons exist in the dentate gyrus (DG) and the olfactory system. Suppressing adult neurogenesis in DG can result in the impairment of discriminating similar memories but not very-different memories. Also, studies found that eliminating adult neurogenesis may link to mood disorders. These results indicate that adult neurogenesis plays a vital role in neural processing. However, how adult neurogenesis contributes to neural information processing remains open. In our recent work, synaptic competition, a process taking part in adult neurogenesis, is essential for pattern separation. Also, we have designed an unsupervised learning rule based on synaptic competition. The learning rule outperforms back-propagation in some classification tasks, e.g., distinguishing digits from the MNIST dataset. Our results suggest that synaptic competition is the key to pattern separation and that competition-based learning could be helpful in machine learning.
每个人大约花三分之一左右的时间在睡眠上。睡眠过程中虽然大脑与外界几乎切断了联系,但是大脑中的神经细胞却忙碌地重放着清醒状态下学习到的信息,从而实现记忆的巩固。然而,睡眠对记忆巩固的作用机理尚不明确,而且很难在活体中进行神经生物学机制的研究。本课题组提出通过构建具有生物物理学特性的丘脑-皮层网络计算模型,探索了不同神经递质对非快动眼睡眠中的脑电信号(比如纺锤波,慢波,纺锤波慢波耦合等)的产生,以及其在记忆巩固任务中的作用和机制。此研究有望将睡眠中的脑电信号作为阿尔兹海默症等认知功能障碍疾病的生物标志物,为期早期预防、诊断及干预提供坚实的理论依据。
摘要:脉冲神经网络在近年由于受到低能耗应用需求而备受关注。端到端反向传播算法给脉冲神经网络训练带来了机会和挑战。本次报告将介绍大规模深度脉冲神经网络如何考虑利用时序信息分布进行端到端学习;同时端到端反向传播算法也给网络带来了鲁棒性挑战,将介绍大规模深度脉冲神经网络如何应对挑战并提高鲁棒性。
情景记忆只能被编码于具有非对称连接权重的神经网络中。在此基础上,我们结合了实验中广泛 观察到的突触可塑性的灵活时间窗口,提出了非对称的高阶联想记忆模型。由于非对称权重带来 的非平衡态动力学,理论研究目前只有很有限的手段。我们从局域动力学的角度进行了切入—— 运用随机矩阵工具计算模型动力学的雅可比矩阵的本征值谱,从中获得局域动力学的关键性质, 并与全局动力学相互联系,最终预测情景记忆的运行。不止于此,这套基于随机矩阵工具的研究 方法也将适用于许多其他神经网络场景。
Traditional Chinese Medicine (TCM), which originated in ancient China with a history of thousands of years, characterizes and addresses human physiology, pathology, and diseases diagnosis and prevention using TCM theories and Chinese herbal products. However, the pharmacological principles in TCM theory, the core treasure house of TCM, have rarely been systematically investigated in a top-down manner, which hinders the modernization and standardization of TCM. In this work, we proposed a novel TCM-based network pharmacology framework to discern general patterns and principles of human disease and predict herb-diseases associations. Specifically, we constructed an integrative database and a pharmacological network of TCM through extensively collecting and cleaning large-scale TCM prescription data from ancient books, modern literature, and existing TCM data resources. Various topological and structural properties of the TCM pharmacological network were systematically characterized to decipher the pharmacological principles of TCM theory. Based on the TCM pharmacological network, we uncovered the human disease-disease relationship and build an in-silico network-based pipeline for the prediction of drug-disease associations. Our work promotes the quantitative underpinning of TCM pharmacological principles, provides a basis for the objectification of the diagnosis and treatment process of Chinese medicine, and paves the way for the knowledge fusion of TCM evidence-based medicine and modern biology.
An Optimal Transport View of Deep Learning
Abstract: In this talk we first introduce an optimal transportation (OT) view of deep learning. Natural datasets have intrinsic patterns, which can be summarized as the manifold distribution principle: the distribution of a class of data is close to a low-dimensional manifold. Deep learning mainly accomplish two tasks: manifold learning and probability distribution transformation. The latter can be carried out using the classical OT method. From the OT perspective, the generator of GAN model computes the OT map, while the discriminator computes the Wasserstein distance between the generated data distribution and the real data distribution; both can be reduced to a convex geometric optimization process. Furthermore, OT theory discovers the intrinsic collaborative—instead of competitive—relation between the generator and the discriminator. Then we give an explanation to mode collapse. By the regularity theory of Monge-Ampere equation the OT map is discontinued if the support of the target distribution is non-convex. But the neural network always represents continue map. This is the reason of mode collapse. Finally based on the above theory we propose three novel models, including generative model AE-OT, super resolutin model OTSR and point cloud upsampling model PU-CycGAN.
Adaptation Accelerating Sampling-based Bayesian Inference in Attractor Neural Networks
The brain performs probabilistic Bayesian inference to interpret the external world. The sampling-based view assumes that the brain represents the stimulus posterior distribution via samples of stochastic neuronal responses. Although the idea of sampling-based inference is appealing, it faces a critical challenge of whether stochastic sampling is fast enough to match the rapid computation of the brain. In this study, we explore how latent feature sampling can be accelerated in neural circuits. Specifically, we consider a canonical neural circuit model called con- tinuous attractor neural networks (CANNs) and investigate how sampling-based inference of latent continuous variables is accelerated in CANNs. Intriguingly, we find that by including noisy adaptation in the neuronal dynamics, the CANN is able to speed up the sampling process significantly. We theoretically derive that the CANN with noisy adaptation implements the efficient sampling method called Hamiltonian dynamics with friction, where noisy adaption effectively plays the role of momentum. We theoretically analyze the sampling performances of the network and derive the condition when the acceleration has the maximum effect. Simulation results validate our theoretical analyses. We further extend the model tocoupled CANNs and demonstrate that noisy adaptation accelerates the sampling of the posterior distribution of multivariate stimuli. We hope that this study enhances our understanding of how Bayesian inference is realized in the brain.
大脑需要执行大量的概率推断任务,采样学派认为神经元的随机发放是在对所需推断的概率进行采样,但是传统的采样算法较慢,很难同大脑的快速反应对应起来,我们的工作提出了一种在连续吸引子网路里实现快速采样算法的机制,网络波包在采样的过程中引入负反馈相当于给其增加了动量项,使其可以累积过往的速度,从而提高采样速度,我们理论证明了这种机制对应了高效的哈密顿采样算法;同时我们证明在多个耦合的吸引子网络中可以实现对多变量的分布式哈密顿采样,我们的实验结果也与理论结果相吻合。
灾难性遗忘目前仍然是深度学习亟待解决的难题之一,即如何让算法在学习新任务的同时,维持历史任务的表现从而实现连续学习。不少来自机器学习界及计算神经科学界的启发式技巧,都能一定程度上缓解遗忘。这里,关于二值神经网络,我们提出了一套统一的学习框架:采用变分框架将网络的训练主体改为权重均值,再通过简单的线性响应关系,在外场空间中进行梯度下降;由此,不确定性将自然地被引入变分框架中,在连续学习的过程中起到重要作用。基于此学习框架,我们首先从统计力学的角度,在单层网络中进行分析;具体来说,我们借用了Franz-Parisi熵的概念构建了网络的势函数,并使用复本方法求解,由此得到的序参量很好地描述了学习的过程,并能预测连续学习的误差。随后,我们在深度网络中运用此学习框架设计算法,并在涉及真实数据的连续学习任务上进行测试,表现出非常惊人的连续学习效果。最后,我们提出的学习框架还与机器学习中经典的EWC(elastic weight consolidation)算法,以及计算神经科学中的权重再可塑性(meta plasticity)相关;因此我们的学习框架证明了基于统计物理模型设计人工智能连续学习算法的有效性。
Image encryption based on hyperchaos combined with different methods
With the development of digital information technology, information security has been widely concerned, and multimedia encryption technology is one of the important technologies to ensure information security. Chaotic sequence cryptography has become an important branch of cryptography as a hot research topic in the field of cryptography. With hyperchaotic system combined with methods like DNA coding and neural network, the image encryption algorithm has the features of complex structure, large key space and strong resistance to attack, which can effectively meet the characteristics of multimedia encryption, so it has a good prospect to be applied to multimedia encryption.
大脑中所接收的信息超过70%都是来自于视觉系统,而视网膜作为心灵之窗,是生物视觉信息处理的第一站,负责对时空中不断变化的可见光进行实时编码。探索视网膜的编码机制对于揭示其它视觉系统的计算原理至关重要。而随着深度学习的发展,人工神经网络已在计算机视觉、自然语言处理等领域取得优越的性能。使用人工神经网络学习视网膜或者初级视皮层响应与输入刺激的映射关系,对了解视网膜等初级视觉系统的工作机制具有巨大优势。视网膜编码模型的研究不仅具有重要的理论研究意义,而且具有实际的工程意义。通过揭示视网膜加工处理信息的工作机理,一方面可以为视觉神经假体的研制提供理论基础;另一方面可以启发设计更加智能的神经形态视觉传感器,例如事件相机与脉冲相机。本报告将着重介绍基于神经网络的单神经节细胞及群体神经节细胞编码模型。这些模型能在学习视觉刺激与神经节细胞响应映射关系的同时,揭示模拟单个神经节神经元功能的最小神经网络系统及群体细胞编码动态刺激的关键因素。
Fractional neural sampling: spatiotemporal probabilistic computations in spiking neural circuits
Neurophysiological recordings have revealed large fluctuations of spiking activity of cortical neurons both over time and across trials, leading to the idea that neural computing in the brain is fundamentally probabilistic. A range of perceptual and cognitive processes have been characterized from the perspective of probabilistic representations and inference. To understand the neural circuit mechanism underlying these probabilistic computations, we develop a theory based on complex spatiotemporal dynamics of neural population activity. By employing a biophysically realistic spiking neural circuit, we show the emergence of spatially structured population activity patterns capturing realistic variability of neural dynamics both in time and in space. These activity patterns exhibit large jumps with fractional order statistics and implement a type of probabilistic computations that we name fractional neural sampling (FNS). We further develop a mathematical model to reveal the algorithmic nature of FNS and its computational advantages for representing multimodal distributions. The FNS theory thus provides a unified account of a diversity of experimental observations of neural spatiotemporal dynamics and perceptual processes, and further provide links between the structure, dynamics, and function of cortical circuits.
为什么看起来如此复杂的神经网络(NN)通常泛化得很好?为了理解这个问题,我们在训练神经网络时发现了一些简单的隐式正则化。首先是神经网络从低频学习到高频的频率原理(频率原则)。其次是参数凝聚,这是非线性训练过程的一个特点,它有效地减小了网络的规模。在此基础上,我们发现了神经网络损失景观的内在嵌入原理以及理解大网络在训练上带来一些优势,并发展了一个秩分析框架,定量地理解一个过度参数化的神经网络需要多少数据量,以便更好地泛化。