CausalAI核心算法:因果森林及其相关研究
导语
在集智俱乐部因果科学读书会第三季,中国科学技术大学统计学博士龚鹤扬介绍了因果树、因果森林及快手的大规模多元因果森林模型。因果树模型的主要贡献是“诚实” (Honest) 的方法,使用非训练样本估计模型的局部参数。相较于传统的“自信” (Adaptive) 的方法,“诚实”的方法有较好的泛化能力。广义随机森林以因果树模型为基础,结合所关注的特定问题,构建算法框架。快手的大规模多元因果森林可以对单一一个模型同时处理任意种干预手段,同时HTE的定义要求各干预手段对应一致的特征子空间。本文是根据此次分享整理的文字稿。
随着“因果革命”在人工智能与大数据领域徐徐展开,作为连接因果科学与深度学习桥梁的因果表征学习,成为备受关注的前沿方向。以往的深度表征学习在数据降维中保留信息并过滤噪音,新兴的因果科学则形成了因果推理与发现的一系列方法。随着二者结合,因果表征学习有望催生更强大的新一代AI。新一季的因果表征学习读书会已经启动,欢迎从事相关研究的各界朋友参与。
研究领域:因果树模型,因果森林

龚鹤扬 | 讲者
贾潍佳 | 整理
邓一雪 | 编辑
1. 因果树
1. 因果树
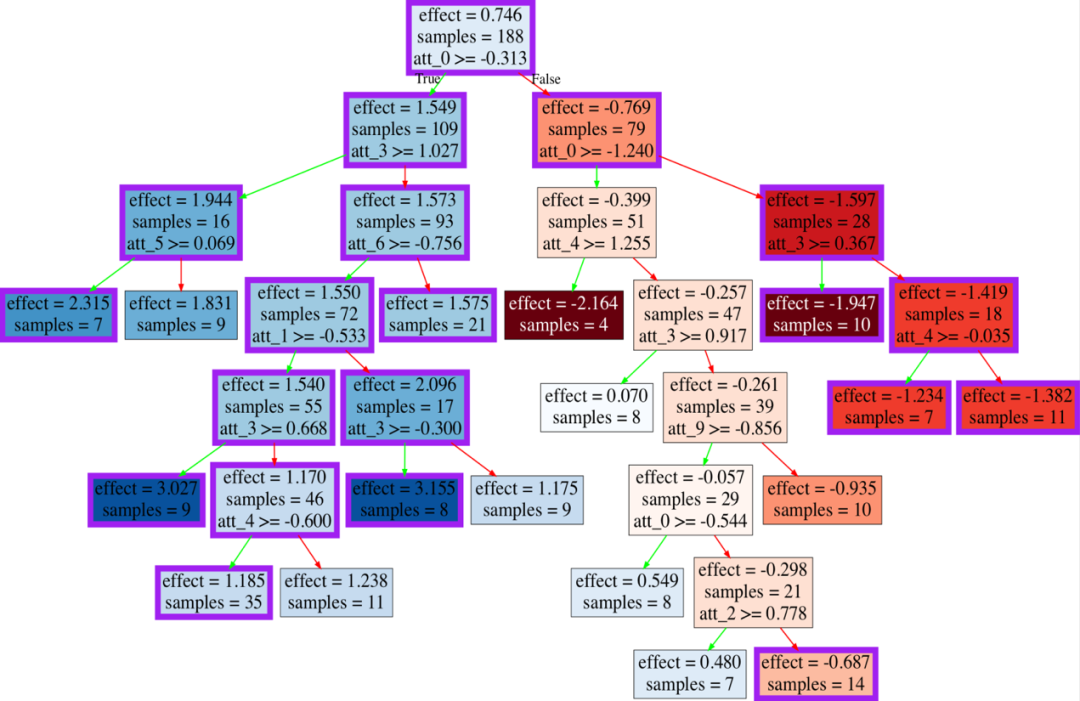

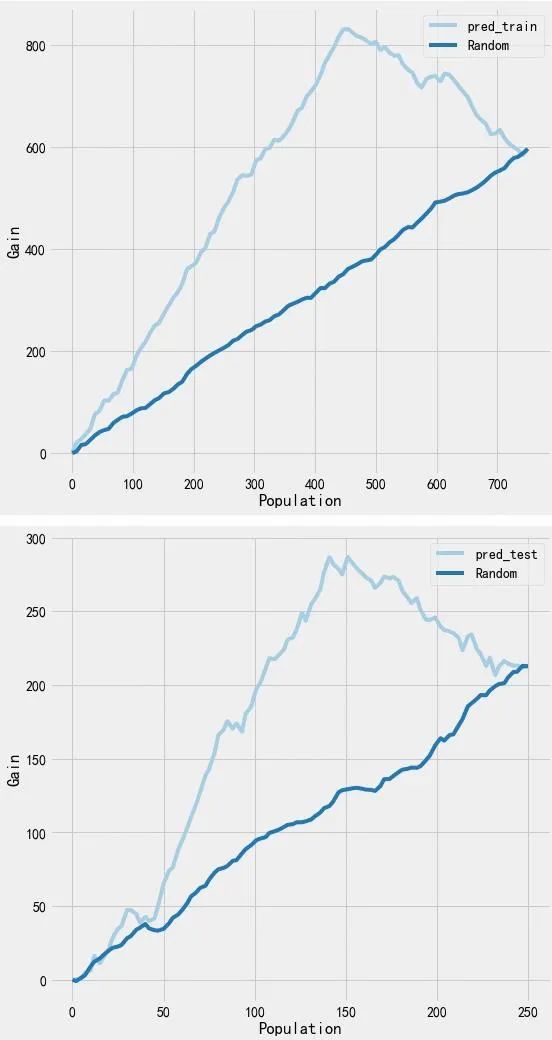
2. 广义随机森林
2. 广义随机森林

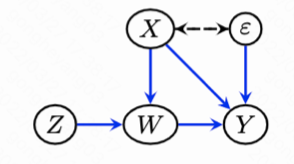

3. 从因果树到广义随机森林
3. 从因果树到广义随机森林
4. 快手的大规模多元因果森林[2]
4. 快手的大规模多元因果森林[2]
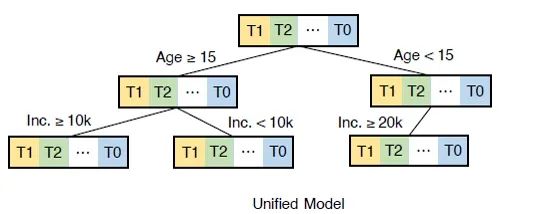
5. 论文推荐
5. 论文推荐
Causal Forest 相关文献
-
Athey S, Imbens G. Recursive partitioning for heterogeneous causal effects[J]. Proceedings of the National Academy of Sciences, 2016, 113(27): 7353-7360.
-
Wager S, Athey S. Estimation and inference of heterogeneous treatment effects using random forests[J]. Journal of the American Statistical Association, 2018, 113(523): 1228-1242.
-
Athey S, Tibshirani J, Wager S. Generalized random forests[J]. The Annals of Statistics, 2019, 47(2): 1148-1178.
-
Athey S, Imbens G W. Machine learning methods that economists should know about[J]. Annual Review of Economics, 2019, 11: 685-725.
-
Tran C, Zheleva E. Learning triggers for heterogeneous treatment effects[C]//Proceedings of the AAAI Conference on Artificial Intelligence. 2019, 33(01): 5183-5190.
-
Evidence-Based Policy Learning(CLeaR 2022 Oral)
-
Nandy P, Yu X, Liu W, et al. Generalized Causal Tree for Uplift Modeling[J]. arXiv preprint arXiv:2202.02416, 2022.
-
Tan X, Chang C C H, Tang L. A tree-based federated learning approach for personalized treatment effect estimation from heterogeneous data sources[J]. arXiv preprint arXiv:2103.06261, 2021.
-
Zeng S, Bayir M A, Pfeiffer III J J, et al. Causal transfer random forest: Combining logged data and randomized experiments for robust prediction[C]//Proceedings of the 14th ACM International Conference on Web Search and Data Mining. 2021: 211-219.
因果之梯: 结构因果模型文献推荐
-
Pearl J. The seven tools of causal inference, with reflections on machine learning[J]. Communications of the ACM, 2019, 62(3): 54-60.
-
Schölkopf B. Causality for machine learning[M]//Probabilistic and Causal Inference: The Works of Judea Pearl. 2022: 765-804.
-
On Pearl’s Hierarchy and the Foundations of Causal Inference
-
Bongers, Stephan, et al. “Foundations of structural causal models with cycles and latent variables.” The Annals of Statistics 49.5 (2021): 2885-2915.
1. Athey S, Imbens G. Recursive partitioning for heterogeneous causal effects[J]. Proceedings of the National Academy of Sciences, 2016, 113(27): 7353-7360.
2. Ai M, Li B, Gong H, et al. LBCF: A Large-Scale Budget-Constrained Causal Forest Algorithm[C]//Proceedings of the ACM Web Conference 2022. 2022: 2310-2319.
因果表征学习读书会启动
随着“因果革命”在人工智能与大数据领域徐徐展开,作为连接因果科学与深度学习桥梁的因果表征学习,成为备受关注的前沿方向。以往的深度表征学习在数据降维中保留信息并过滤噪音,新兴的因果科学则形成了因果推理与发现的一系列方法。随着二者结合,因果表征学习有望催生更强大的新一代AI。
集智俱乐部组织以“因果表征学习”为主题、为期十周的读书会,聚焦因果科学相关问题,共学共研相关文献。欢迎从事因果科学、人工智能与复杂系统等相关研究领域,或对因果表征学习的理论与应用感兴趣的各界朋友报名参与。集智俱乐部已经组织三季“因果科学”读书会,形成了超过千人的因果科学社区,因果表征学习读书会是其第四季,现在加入读书会即可参与因果社区各类线上线下交流合作。
推荐阅读
-
因果科学算法、框架、数据集汇总 -
大数据因果推断:数据驱动式学习下的因果混淆去偏算法 -
前沿算法:如何利用群论进行深度学习下的因果特征解耦 -
《张江·复杂科学前沿27讲》完整上线! -
成为集智VIP,解锁全站课程/读书会 -
加入集智,一起复杂!
点击“阅读原文”,报名读书会