第三代神经网络模型:面向AI应用的脉冲神经网络
导语

1. 神经元模型
2. 编码方式
3. 学习算法
4. 网络结构
5. 总结和展望
1. 神经元模型
1. 神经元模型
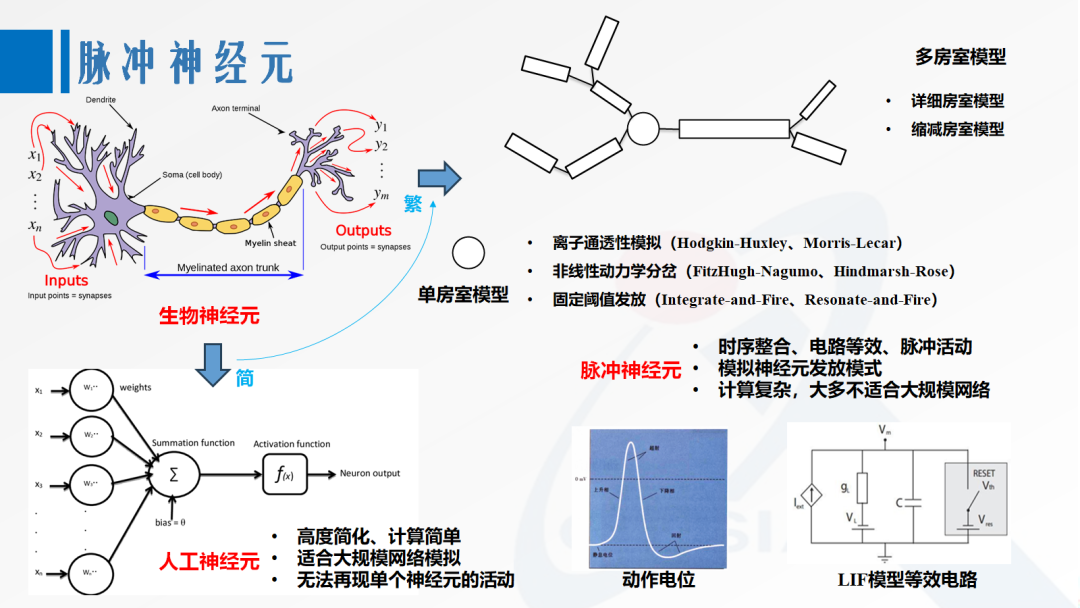
[1] Dayan P, Abbott L F. Theoretical neuroscience: computational and mathematical modeling of neural systems [M]. MIT press, 2005.
[2] Izhikevich E M. Simple model of spiking neurons [J]. IEEE transactions on neural networks, 2003, 14(6): 1569-1572. [3] Perez-Nieves N, Leung V C H, Dragotti P L, et al. Neural heterogeneity promotes robust learning[J]. Nature communications, 2021, 12(1): 5791.

2. 编码方式
2. 编码方式
[4] Adrian E D, Zotterman Y. The impulses produced by sensory nerve-endings: Part ii. the response of a single end-organ [J]. The Journal of physiology, 1926, 61(2): 151. [5] VanRullen R, Guyonneau R, Thorpe S J. Spike times make sense [J]. Trends in neurosciences, 2005, 28(1): 1-4.

[6] Pouget A, Dayan P, Zemel R. Information processing with population codes [J]. Nature reviews neuroscience, 2000, 1(2): 125-132.
[7] Olshausen B A, Field D J. Sparse coding of sensory inputs [J]. Current opinion in neurobiology, 2004, 14(4): 481-487. [8] Zhang D, Zhang T, Jia S, et al. Multi-sacle dynamic coding improved spiking actor network for reinforcement learning[C]//Proceedings of the AAAI Conference on Artificial Intelligence. 2022, 36(1): 59-67.
[9] Panzeri S, Brunel N, Logothetis N K, et al. Sensory neural codes using multiplexed temporal scales [J]. Trends in neurosciences, 2010, 33(3): 111-120.
3. 学习算法
3. 学习算法
[10] Do H. The organization of behavior [J]. New York, 1949. [11] Markram H, Lübke J, Frotscher M, et al. Regulation of synaptic efficacy by coincidence of postsynaptic aps and epsps [J]. Science, 1997, 275(5297): 213-215.
[12] Cao Y, Chen Y, Khosla D. Spiking deep convolutional neural networks for energy-efficient object recognition [J]. International journal of computer vision, 2015, 113: 54-66.
[13] Wu Y, Deng L, Li G, et al. Spatio-temporal backpropagation for training high-performance spiking neural networks [J]. Frontiers in neuroscience, 2018, 12: 323875.
[14] Frémaux N, Gerstner W. Neuromodulated spike-timing-dependent plasticity, and theory of three-factor learning rules [J]. Frontiers in neural circuits, 2016, 9: 85.
[15] Zhang T, Cheng X, Jia S, et al. A brain-inspired algorithm that mitigates catastrophic forgetting of artificial and spiking neural networks with low computational cost[J]. Science Advances, 2023, 9(34): eadi2947.
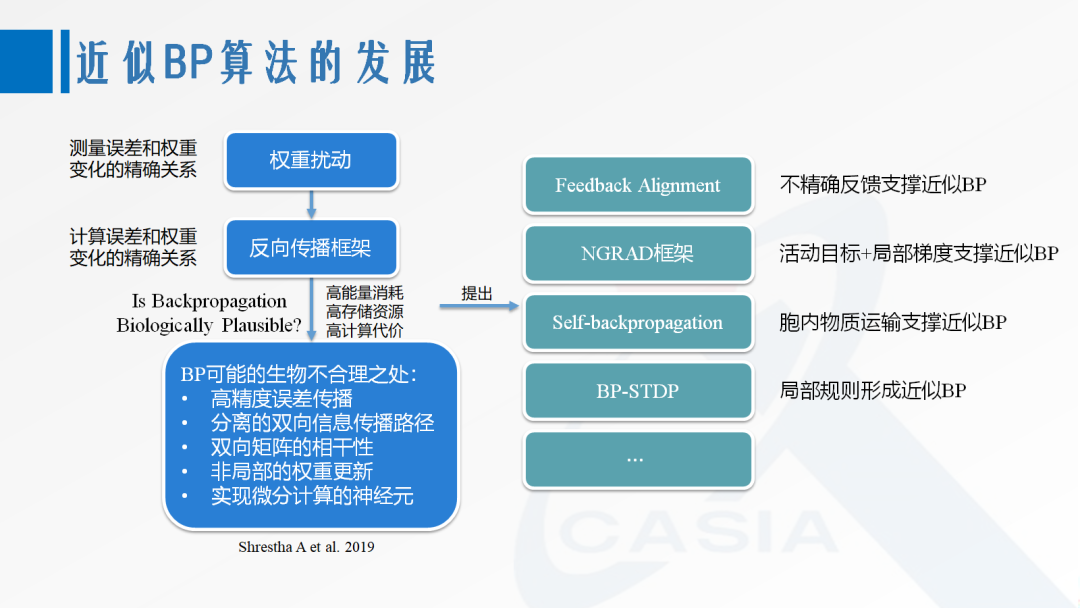
[16] Lillicrap T P, Cownden D, Tweed D B, et al. Random synaptic feedback weights support error backpropagation for deep learning [J]. Nature communications, 2016, 7(1): 13276. [17] Lillicrap T P, Santoro A, Marris L, et al. Backpropagation and the brain [J]. Nature reviews neuroscience, 2020, 21(6): 335-346. [18] Zhang T, Cheng X, Jia S, et al. Self-backpropagation of synaptic modifications elevates the efficiency of spiking and artificial neural networks[J]. Science advances, 2021, 7(43): eabh0146. [19] Tavanaei A, Maida A. BP-STDP: Approximating backpropagation using spike timing dependent plasticity[J]. Neurocomputing, 2019, 330: 39-47.

[20] Stevens C F, Wang Y. Facilitation and depression at single central synapses [J]. Neuron, 1995, 14(4): 795-802.
4. 网络结构
4. 网络结构
[21] Cheng X, Hao Y, Xu J, et al. LISNN: Improving spiking neural networks with lateral interactions for robust object recognition[C]//IJCAI. 2020: 1519-1525.
[22] Hasani R, Lechner M, Amini A, et al. A natural lottery ticket winner: Reinforcement learning with ordinary neural circuits[C]//International Conference on Machine Learning. PMLR, 2020: 4082-4093.
[23] Zhang D, Zhang T, Jia S, et al. Multi-sacle dynamic coding improved spiking actor network for reinforcement learning[C]//Proceedings of the AAAI Conference on Artificial Intelligence. 2022, 36(1): 59-67.
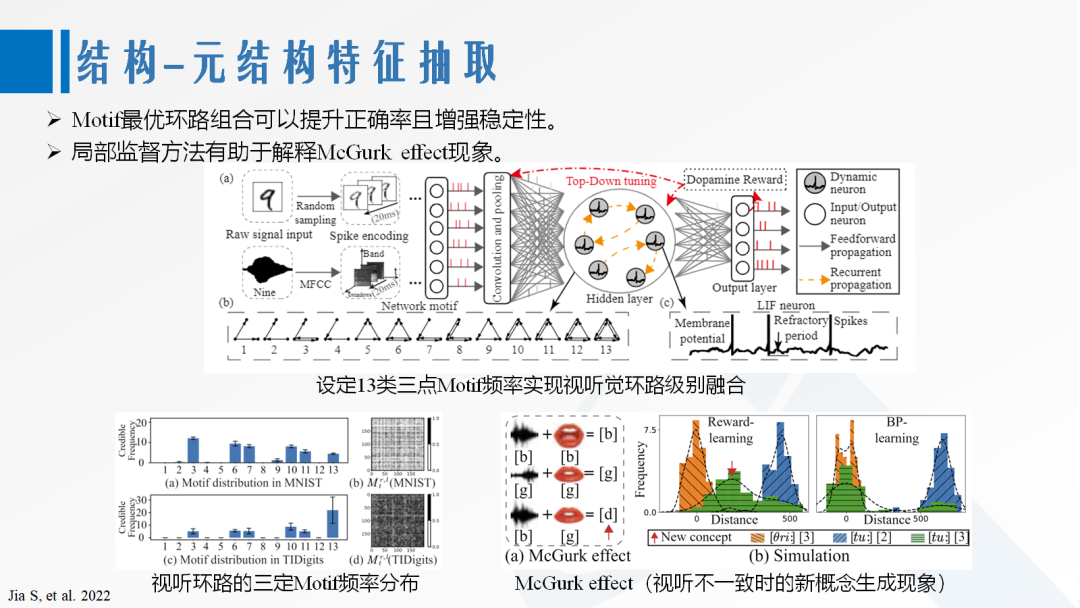
5. 总结和展望
5. 总结和展望
作者简介
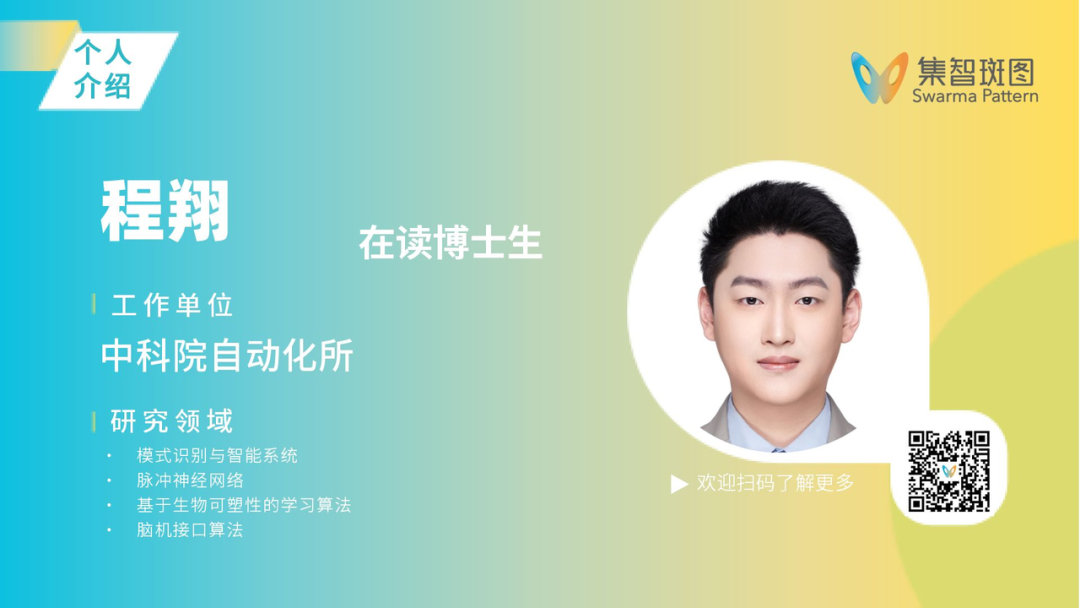
计算神经科学读书会
详情请见:计算神经科学读书会启动:从复杂神经动力学到类脑人工智能
点击“阅读原文”,报名读书会