统计物理与信息论视角下,结构化噪声与神经网络初始化研究|周一直播·AI by Complexity读书会
导语
主题一:破解数据噪声难题:结构化噪声下PCA的信息论极限
主题一:破解数据噪声难题:结构化噪声下PCA的信息论极限
内容大纲
-
前置知识
-
主成分分析介绍
-
I-MMSE关系与已知的渐近行为
-
噪声结构与数学分析
-
副本方法与副本对称自由熵
-
Nishimori 恒等式和鞍点方程
-
自适应TAP方法
-
结果分析
-
Fundamental limits in structured principal component analysis and how to reach them, J. Barbier, F.Camilli, M. Mondelli, M. S ́aenz, Proceedings of the National Academy of Sciences, 120 (30) e2302028120 (2023)
-
Information limits and Thouless-Anderson-Palmer equations for spiked matrix models with structured noise, J. Barbier, F. Camilli, M. Mondelli, Y. Xu, arXiv preprint arXiv:2405.20993, 2024.
-
Random matrix methods for high-dimensional machine learning models, A. Bodin, PhD Thesis, EPFL, doi.org/10.5075/epfl-thesis-10524, 2024
-
Adaptive and Self-Averaging Thouless-Anderson-Palmer Mean-Field Theory for Probabilistic Modeling, M. Opper, O. Winther, Physical Review E 64 5 (2001)
-
A First Course in Random Matrix Theory: For Physicists, Engineers and Data Scientists, M. Potters, J.-P. Bouchaud, Cambridge University Press, 2020
-
Optimality of Approximate Message Passing Algorithms for Spiked Matrix Models with Rotationally Invariant Noise, R. Dudeja, S. Liu, J. Ma, arXiv preprint arXiv:2405.18081, 2024
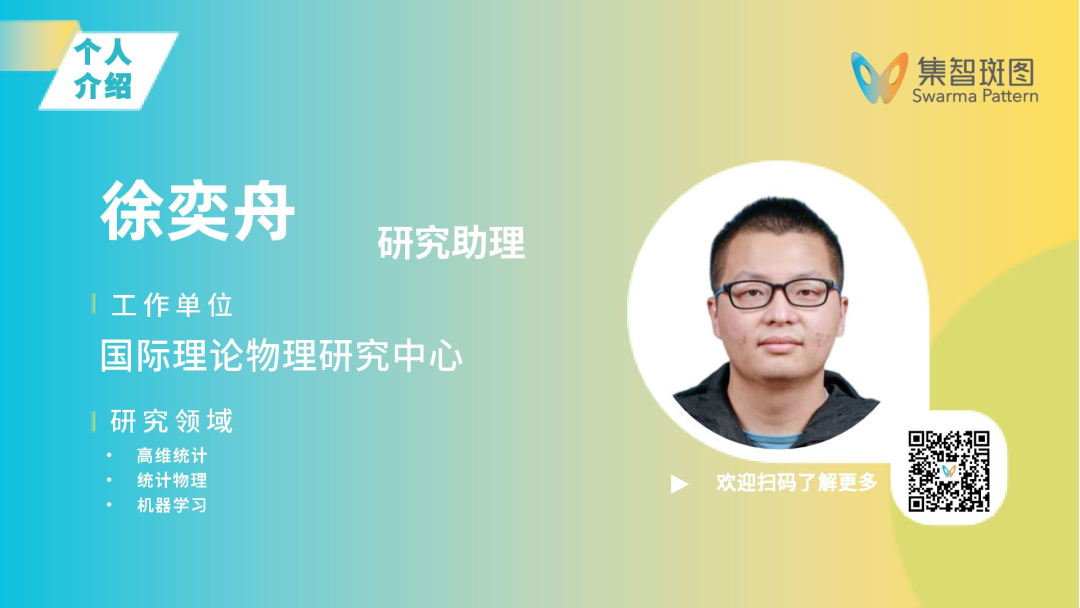
主题二:神经网络的初始化和信息论
主题二:神经网络的初始化和信息论
内容简介
在深度学习中,神经网络可以视为输入数据和其潜在表示之间的含噪信道,这个视角将我们引向了对神经网络的信息传输性质的研究。我们研究发现神经网络可以在初始化时就朝最优信道发展。
分享大纲
1. 基础知识
a. 随机初始化神经网络:几何角度的理论与实验结果
b. 互信息:在神经网络中的应用与测量
1. Weng, Kangyu, et al. "Statistical physics of deep neural networks: Initialization toward optimal channels." Physical Review Research 5.2 (2023): 023023.
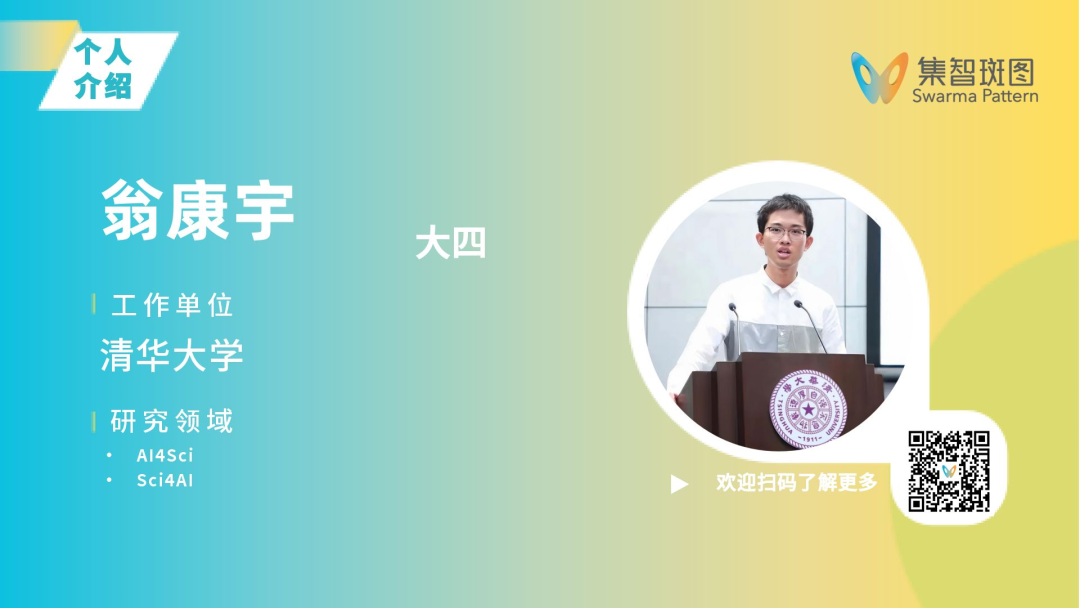
直播信息
直播信息
时间:2024年7月1日(周一)晚20:00-22:00
报名参与读书会:
报名成为主讲人
读书会成员均可以在读书会期间申请成为主讲人。主讲人作为读书会成员,均遵循内容共创共享机制,可以获得报名费退款,并共享本读书会产生的所有内容资源。详情请见:AI by Complexity 读书会启动:复杂性怎样量化和驱动下一代AI系统
AI By Complexity读书会招募中