本周的「因果涌现」读书会将由约翰·霍普金斯大学在读研究生荣英淇介绍因果涌现理论在脑网络中的应用。回顾脑领域与因果涌现研究密切相关的学者,Luppi 等人主要通过信息分解工具探索意识的机制,Varley 等人则通过信息论指标衡量脑功能。此次读书会希望从这两种视角探讨因果涌现理论在脑领域应用中的可能问题。
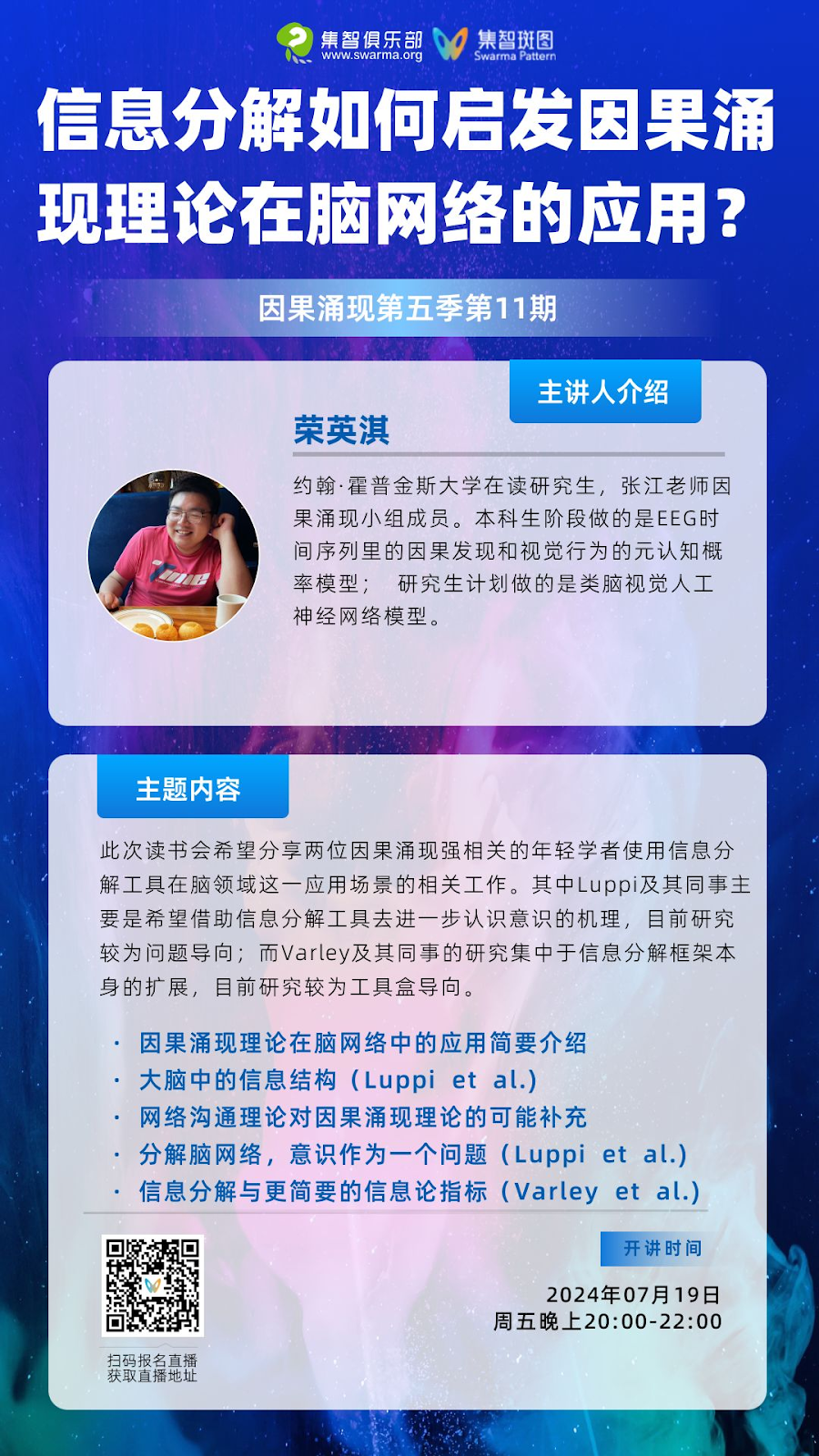
因果涌现读书会已经进展到第5季末尾,来自不同领域背景的老师同学已经有许多深入的讨论和产出。不少关键文献也已经经过了反复讨论、阅读和推敲,其中比较具有代表性的分别是:Erik Hoel 及其同事结合干预主义因果(interventionist causality)和有效信息提出因果涌现框架[1-2],和 Fernando Rosas 及其同事结合转移熵和信息分解对因果涌现进行了再定义[3-4]。
但依旧可能有许多对因果涌现乃至涌现这一概念保持质疑的老师同学会发问,其应用点在哪里?如果涌现这一大问题能够被解决,那么我们应该期待许多子问题或小问题也能被阐述得更清楚。此次读书会的核心目的并非去试图去打消质疑,而是同样作为一名感到困惑挣扎想探索方向的学生,和大家分享两位因果涌现方向的年轻学者(直接参与过相关工作)使用信息分解工具在脑领域这一应用场景中的相关工作。
其中 Luppi 及其同事主要是希望借助信息分解工具进一步认识意识的机理,目前研究较为问题导向[5-6], ;而Varley及其同事的研究集中于信息分解框架本身的扩展,目前研究较为工具盒导向[10,12]。所以,前者近期发表的综述可能会在大的应用场景上有更清晰的阐述,而后者会在具体的信息论指标上有所发展。
此次读书会希望通过对大的科学故事和具体的信息论指标的讨论,来一同追寻是否能从中获得启发(为什么脑领域和因果涌现关联大?可见下图或《脑与涌现的联系:从协同核到因果涌现》),推敲因果涌现理论应用于脑领域的可能问题,比如:
(1)对于Hoel的框架,确定性和简并性在脑网络里意味着什么?是否能从网络沟通模型(network communication models)得到启发?(《量化因果涌现表明:宏观可以战胜微观》)
(2)对于Rosas的框架,如何解决或回避信息分解本身的计算复杂度?哪些更为简要的信息指标能对脑网络进行衡量?(《量化涌现:信息论方法识别多变量数据中的因果涌现》)
(3)对于NIS及其扩展框架,通过结合网络神经科学的知识,能否进一步改善对脑网络粗粒化和动力学拟真的策略?(《什么是涌现?人工智能给你答案》)
1.因果涌现相关学者网络简要介绍
2.因果涌现理论在脑网络中的应用简要介绍
3. 大脑中的信息结构(Luppi et al.)
4. 网络沟通理论对因果涌现理论的可能补充
5. 分解脑网络,意识作为一个问题(Luppi et al.)
6. 信息分解与更简要的信息论指标(Varley et al.)
脑网络 Brain Network
信息分解 Information Decomposition
因果涌现 Causal emergence
协同核 Synergistic core
局部信息分解 Partial information decomposition, PID
局部熵分解 Partial entropy decomposition, PED
网络沟通模型 Network communication models
荣英淇,约翰·霍普金斯大学在读研究生,张江老师因果涌现小组成员。本科阶段做的是EEG时间序列里的因果发现和视觉行为的元认知概率模型;研究生阶段做的是不同视觉系统的表征对齐。
时间:2024年7月19日(本周五)晚20:00-22:00
斑图链接:https://pattern.swarma.org/study_group_issue/693
扫码参与因果涌现读书会,加入群聊,获取系列读书会回看权限,加入因果涌现社区,与社区的一线科研工作者沟通交流,共同推动因果涌现这一前沿领域的发展。
报名成为主讲人:
1. Hoel EP, Albantakis L, Tononi G. Quantifying causal emergence shows that macro can beat micro. Proc Natl Acad Sci U S A. 2013 Dec 3;110(49):19790-5. doi: 10.1073/pnas.1314922110. Epub 2013 Nov 18. PMID: 24248356; PMCID: PMC3856819.
2. Tononi, G., Sporns, O. Measuring information integration. BMC Neurosci 4, 31 (2003). https://doi.org/10.1186/1471-2202-4-31
3. Rosas, Fernando E., et al. “Reconciling emergence: An information-theoretic approach to identify causal emergence in multivariate data.” PLoS computational biology 16.12 (2020): e1008289.
4. Mediano PAM, Rosas FE, Luppi AI, Jensen HJ, Seth AK, Barrett AB, Carhart-Harris RL, Bor D. 2022 Greater than the parts: a review of the information decomposition approach to causal emergence. Phil. Trans. R. Soc. A 380: 20210246. https://doi.org/10.1098/rsta.2021.024
5. Luppi, A. I., Rosas, F. E., Mediano, P. A. M., Menon, D. K., & Stamatakis, E. A. (2024). Information decomposition and the informational architecture of the brain. Trends in Cognitive Sciences, 28(4), 352–368. https://doi.org/10.1016/j.tics.2023.11.005
6. Luppi, A. I., Rosas, F. E., Mediano, P. A. M., Demertzi, A., Menon, D. K., & Stamatakis, E. A. (2024). Unravelling consciousness and brain function through the lens of time, space, and information. Trends in Neurosciences, 47(7), 551-568. https://doi.org/10.1016/j.tins.2024.05.007
7. Betzel, R. F., & Bassett, D. S. (2017). Multi-scale brain networks. NeuroImage, 160, 73–83. https://doi.org/10.1016/j.neuroimage.2016.11.006
8. P.A.M. Mediano, et al. Towards an extended taxonomy of information dynamics via integrated information decomposition. arXiv (2021). http://doi.org/10.48550/arxiv.2109.13186
9. Luppi, A. I., Mediano, P. A. M., Rosas, F. E., Allanson, J., Pickard, J. D., Carhart-Harris, R., Williams, G. B., Craig, M. M., Finoia, P., Owen, A. M., Naci, L., Menon, D. K., Bor, D., & Stamatakis, E. A. (2024). A synergistic workspace for human consciousness revealed by integrated information decomposition. *eLife, 88173. https://doi.org/10.7554/eLife.88173
10. Varley, T. F. (2024). Generalized decomposition of multivariate information. PLOS ONE, 19(2), e0297128. https://doi.org/10.1371/journal.pone.0297128
11. Yuan, B., Zhang, J., Lyu, A., Wu, J., Wang, Z., Yang, M., Liu, K., Mou, M., & Cui, P. (2024). Emergence and Causality in Complex Systems: A Survey of Causal Emergence and Related Quantitative Studies. Entropy, 26(1), 108. https://doi.org/10.3390/e26020108
12. Varley, T. F., Pope, M., Puxeddu, M. G., Faskowitz, J., & Sporns, O. (2023). Partial entropy decomposition reveals higher-order information structures in human brain activity. Proceedings of the National Academy of Sciences, 120(30), e2300888120. https://doi.org/10.1073/pnas.2300888120
13. A.M. Proca, et al. Synergistic information supports modality integration and flexible learning in neural networks solving multiple tasks. arXiv (2022) Published online October 6, 2022. https://doi.org/10.48550/arXiv.2210.02996
14. Amico, E., Abbas, K., Duong-Tran, D. A., Tipnis, U., Rajapandian, M., Chumin, E., Ventresca, M., Harezlak, J., & Goñi, J. (2021). Toward an information theoretical description of communication in brain networks. Network Neuroscience, 5(3), 646–665. https://doi.org/10.1162/netn_a_00185
15. Huang, Z., Mashour, G. A., & Hudetz, A. G. (2023). Functional geometry of the cortex encodes dimensions of consciousness. Nature Communications, 14, 72. https://doi.org/10.1038/s41467-022-35764-7
16. Luppi, A. I., Vohryzek, J., Kringelbach, M. L., Mediano, P. A. M., Craig, M. M., Adapa, R., … & Stamatakis, E. A. (2023). Distributed harmonic patterns of structure-function dependence orchestrate human consciousness. Communications Biology, 6, 117. https://doi.org/10.1038/s42003-023-04474-1
17. Seth, A. K., Barrett, A. B., & Barnett, L. (2011). Causal density and integrated information as measures of conscious level. Philosophical Transactions of the Royal Society A: Mathematical, Physical and Engineering Sciences, 369(1942), 3748–3767. https://doi.org/10.1098/rsta.2011.0079
18. Zhang, Jiang & Liu, Kaiwei. (2022). Neural Information Squeezer for Causal Emergence. Entropy. 2023. 10.3390/e25010026.
19. Yang, Mingzhe, et al. “Finding emergence in data: causal emergence inspired dynamics learning.” arXiv preprint arXiv:2308.09952 (2023).
20. Paul L. Williams,Randall D. Beer: Nonnegative Decomposition of Multivariate Information ,arXiv:1004.2515,2010
跨尺度、跨层次的涌现是复杂系统研究的关键问题,生命起源和意识起源这两座仰之弥高的大山是其代表。从2021年夏天至今,集智俱乐部已经陆续举办了四季「因果涌现」读书会,系统梳理了因果涌现理论的发展脉络,深入探讨了信息整合与信息分解的本质,并探索了在生物网络、脑网络、机器学习等跨学科领域的应用。此次因果涌现读书会第五季将追踪因果涌现领域的前沿进展,展示集智社区成员的原创性工作,希望探讨因果涌现理论、复杂系统的低秩表示理论、本征微观态理论之间的相通之处,对复杂系统的涌现现象有更深刻的理解。读书会从2024年4月19日开始,每周五晚20:00-22:00进行,持续时间预计8-10周。欢迎感兴趣的朋友报名参与!
作为北师大系统科学学院的教授,以及集智俱乐部、集智学园的创始人,集智科学研究中心院长,张江从2003年开始,就长期从事有关复杂系统建模的工作。近年来,张江带领着北师大的研究组开始聚焦在基于新兴AI技术进行基于数据驱动的自动建模研究,并立志破解复杂系统的涌现之谜。我们希望可以有对复杂系统自动建模领域有热情,且认可这个领域发展前景的朋友一起来合作,促进这一领域的快速发展。我们希望这个叫做“ Complexity AI ”,中文叫做“复杂AI次方”的开放实验室,能够真正实现思想共享、资源共享、跨学科交叉,共同为复杂系统自动建模而奋进。
详情请见:“复杂 AI 次方”开放实验室招募,挑战“涌现”难题
点击“阅读原文”,报名读书会