混合型社会:设计自组织系统集群行为的挑战及前景 | 复杂性文摘

为什么国家卫生研究院(NIH)应该用抽签替换同行评审?
如何通过稀疏识别从数据中发掘非线性动力系统的控制方程?
混合型社会是否是一种由不同组件组成的自组织的集群系统?
集智复杂文摘翻译小组为你呈现新鲜的研究内容!

为什么国家卫生研究院(NIH)应该用抽签替换同行评审(Why the National Institutes of Health Should Replace Peer Review With a Lottery)
PSMAGApril 11, 3:39 PM
BY Michael White
(Translated by – 李昉)
edited by 傅渥成
用抽签决定基金的主要好处是减少对同行评审排名的依赖,国家卫生研究院(NIH)将有更多的空间来解决这个紧急问题。适当设计的抽签系统可以帮助消除微小但持续的性别差距,以及获取基金方面巨大的种族差异,并给予 NIH 工作人员在选择具有代表性的研究计划时更多的回旋余地。重要的是,对于抽签,大多数科学家已经坦然承认,尽管根据价值作出决策取得很多成绩,但同行评审是一个比我们理解的更具随机性的过程。
A major advantage of using a funding lottery would be that, by reducing its reliance on peer-review rankings, the NIH would have more room to address the urgent problem of bias. A suitably designed lottery system could help eliminate the small but persistent gender gap, and the larger racial gap in the funding it awards, by giving NIH officers more leeway to include a representative set of proposals. And importantly, a lottery would be an honest acknowledgement of what most scientists already sense: that, despite its reputation for basing decisions on merit, peer review is a much more random process than we would like to admit.
原文链接:https://psmag.com/why-the-national-institutes-of-health-should-replace-peer-review-with-a-lottery-41d09bcce5d8?gi=cf38b8f1f21d

Robotics and AI, April 11, 5:44 PM
BY Heiko Hamann, Yara Khaluf, Jean Botev, Mohammad Divband Soorati, Eliseo Ferrante, Oliver Kosak, Jean-Marc Montanier, Sanaz Mostaghim, Richard Redpath, Jonathan Timmis, Frank Veenstra, Mostafa Wahby, Aleš Zamuda
(Translated by -xiaomuqiu)
edited by 傅渥成
混合型社会是一种由不同组件组成的自组织的集群系统,例如,天然和人工部分(生物混合系统)或人类与技术系统及通过技术系统相互作用(社会-技术系统)。许多不同学科所采用的研究方法和研究体系都与设计混合型社会有密切关联。这些学科间强有力的合作可以使方法得到重新使用,并创造显著的协同效应。我们明确了设计自组织混合型社会的三大领域的挑战。首先,我们明确了形式化的挑战。即迫切需要一个通用模型来描述和比较不同的混合型社会集群。其次,我们明确了系统设计的挑战。即我们需要从系统的形式化规范为起点,建立一个整合性的设计过程。第三,我们明确了跨学科的挑战。即自组织混合社会当前研究横跨许多不同的领域,因此我们需要将其方法在不同学科的交叉中进行综合再利用。最后,我们对这一高潜力领域未来的研究方法提出了观点。
Hybrid societies are self-organizing, collective systems, which are composed of different components, for example, natural and artificial parts (bio-hybrid) or human beings interacting with and through technical systems (socio-technical). Many different disciplines investigate methods and systems closely related to the design of hybrid societies. A stronger collaboration between these disciplines could allow for re-use of methods and create significant synergies.We identify three main areas of challenges in the design of self-organizing hybrid societies. First, we identify the formalization challenge.There is an urgent need for a generic model that allows a description and comparison of collective hybrid societies. Second, we identify the system design challenge. Starting from the formal specification of the system, we need to develop an integrated design process. Third, we identify the challenge of interdisciplinarity. Current research on self-organizing hybrid societies stretches over many different fields and hence requires the re-use and synthesis of methods at intersections between disciplines. We then conclude by presenting our perspective for future approaches with high potential in this area.
原文链接:
http://journal.frontiersin.org/article/10.3389/frobt.2016.00014/full

PNASApril 13, 3:45 PM
BY Steven L. Brunton, Joshua L. Proctor, and J. Nathan Kutz
(Translated by -Darthy)
(Edited by 唐璐)
如何从数据中提取控制方程是很多科学和工程领域面临的一个主要问题。在很多领域中,数据很多,模型却难以捉摸,例如气候科学、神经科学、生态学、金融和流行病学。在本文中,我们将稀疏技术和机器学习与非线性动力系统结合起来,从带噪声的观测数据中挖掘控制方程。对模型结构的唯一假设为:系统的动力学仅由很少的几个重要因素决定,因此方程在可能函数的空间中是稀疏的;这一假设在一定条件下对很多物理系统中都能成立。具体来说,我们使用稀疏回归来判定精确表示数据所需的动力学控制方程的最少因素。该方法所生成的精简模型能够在模型的精确性和复杂性之间进行平衡,避免过度拟合。我们用各种问题上验证了该算法的有效性,从简单的标准系统,包括线性和非线性振子系统,以及混沌洛伦兹系统,到障碍物后面形成的涡流。流体的例子证明了这个方法发现系统背后的动力学的能力,专家们曾花了近30年时间才解决这个问题。我们的研究还表明,该方法可以推广到参数化系统、时变系统或受到外力的系统中。
Extracting governing equations from data is a central challenge in many diverse areas of science and engineering. Data are abundant whereas models often remain elusive, as in climate science, neuroscience, ecology, finance, and epidemiology, to name only a few examples. In this work, we combine sparsity-promoting techniques and machine learning with nonlinear dynamical systems to discover governing equations from noisy measurement data. The only assumption about the structure of the model is that there are only a few important terms that govern the dynamics, so that the equations are sparse in the space of possible functions; this assumption holds for many physical systems in an appropriate basis. In particular, we use sparse regression to determine the fewest terms in the dynamic governing equations required to accurately represent the data. This results in parsimonious models that balance accuracy with model complexity to avoid overfitting. We demonstrate the algorithm on a wide range of problems, from simple canonical systems, including linear and nonlinear oscillators and the chaotic Lorenz system, to the fluid vortex shedding behind an obstacle. The fluid example illustrates the ability of this method to discover the underlying dynamics of a system that took experts in the community nearly 30 years to resolve. We also show that this method generalizes to parameterized systems and systems that are time-varying or have external forcing.
原文链接:http://www.pnas.org/content/113/15/3932.abstract
(图片均来自于网络)
复杂文摘翻译小组翻译作品集锦
复杂文摘翻译第2期
编辑:wangting
让苹果砸得更猛烈些吧!
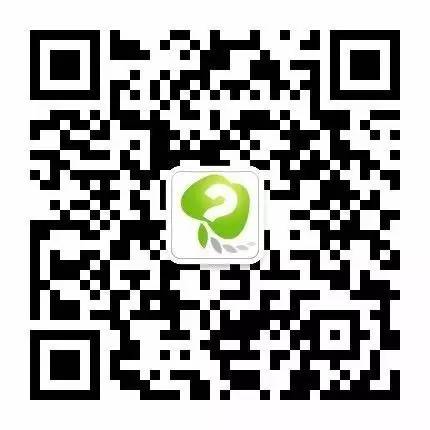
长按识别二维码,关注集智Club,
让我们离科学探索更近一步。
始发于微信公众号: 集智俱乐部