黄海平:神经网络的统计力学课程 | 50人免费名额
导语
为了帮助学生学习统计力学的基本原理及其在理解神经网络内部工作原理的应用,中山大学教授、PMI Lab的黄海平老师组织了《神经网络的统计力学》在线课程,暂定于2022年9月17日起每周六14:30-16:00(节假日除外)进行,课程从2022年9月持续到2023年6月。

课程背景
课程背景
课程涉及黄海平老师最新出版的书籍《Statistical Mechanics of Neural Networks》的大部分内容,目标是学习统计力学的基本原理及其在理解神经网络内部工作原理的应用。
负责人介绍
负责人介绍
PMI Lab:https://www.labxing.com/hphuang2018
正式学员申请要求与流程
正式学员申请要求与流程
学习名额:课程招收50名正式学员。正式学员受课程负责人黄海平老师进行严格的课程学习管理,完成课程作业、课程小项目论文等。
培养目标:培养一批有望在神经网络理论深耕的青年学者(侧重学生)
申请人要求:对统计⼒学、神经⽹络及脑科学交叉感兴趣的研究⽣/本科⽣/博⼠后均可报名课程,需具备⾼等数学、概率论、线性代数、python/C语⾔、统计⼒学基础(例如教科书)。有神经⽹络或计算神经科学知识者优先。
申请截⽌⽇期:2022年8⽉31⽇(需附简历⾄huanghp7@mail.sysu.edu.cn)。
申请成功学员福利:本课程会由集智学园进行免费直播,收费录播。但是经黄海平老师筛选,被成功录取的正式学员可免费获取视频录播权限。具体在确认学员名单后,会统一开通。
课程回放
课程回放
本课程由集智学园进行免费直播,收费录播。课程定价:499,时间周期在 2022.09-2023.06 持续更新。从9月开始更新,现在处于预售阶段。未成为正式学员的同学,可付费报名录播,参与学习。
课程时间:从2022年9月17日起,每周六 14:30-16:00(节假日除外)
扫码付费报名课程
-
扫码付费; -
课程页面添加学员登记表,添加助教微信入群; -
课程可开发票。
书籍介绍
书籍介绍
本书涵盖了用于理解神经网络原理的必要统计力学知识,包括复本方法、空腔方法、平均场近似、变分法、随机能量模型、Nishimori条件、动力学平均场理论、对称性破缺、随机矩阵理论等,同时详细描述了监督学习、无监督学习、联想记忆网络、感知器网络、随机循环网络等神经网络及其功能的物理模型以及解析理论。本书通过简洁的模型展示了神经网络原理的数学美和物理深度,并介绍了相关历史和展望未来研究的重要课题,可供对神经网络原理感兴趣的学生、研究人员以及工程师阅读参考。
关键词:统计力学,神经网络,机器学习,随机矩阵,非线性动力学
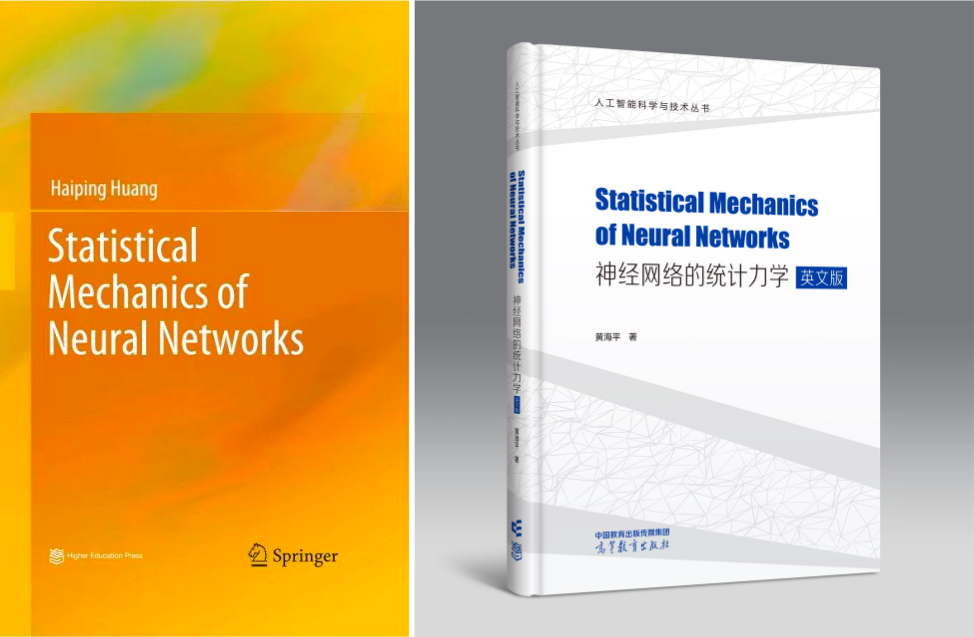
Statistical Mechanics of Neural Networks
本书海外版由 Springer 发行,目前可在 Amazon 平台购买。国内由高教出版社发行,预计8月中旬书籍上架,可在国内各大平台购买。
书籍目录(详版):
Chapter 2: Spin Glass Models and Cavity Method
-
2.1 Multi-spin Interaction Models
-
2.2 Cavity Method
-
2.3 From Cavity Method to Message Passing Algorithms
Chapter 3: Variational Mean-Field Theory and Belief Propagation
-
3.1 Variational Method
-
3.2 Variational Free Energy
-
3.3 Mean-Field Inverse Ising Problem
Chapter 4: Monte Carlo Simulation Methods
-
4.1 Monte Carlo Method
-
4.2 Importance Sampling
-
4.3 Markov Chain Sampling
-
4.4 Monte Carlo Simulations in Statistical Physics
Chapter 5: High-Temperature Expansion
-
5.1 Statistical Physics Setting
-
5.2 High-Temperature Expansion
-
5.3 Properties of the TAP Equation
Chapter 6: Nishimori Line
-
6.1 Model Setting
-
6.2 Exact Result for Internal Energy
-
6.3 Proof of No RSB Effects on the Nishimori Line
Chapter 7: Random Energy Model
-
7.1 Model Setting
-
7.2 Phase Diagram
Chapter 8: Statistical Mechanical Theory of Hopfifield Model
-
8.1 Hopfifield Model
-
8.2 Replica Method
-
8.3 Phase Diagram
-
8.4 Hopfifield Model with Arbitrary Hebbian Length
Chapter 9: Replica Symmetry and Replica Symmetry Breaking
-
9.1 Generalized Free Energy and Complexity of States
-
9.2 Applications to Constraint Satisfaction Problems
-
9.3 More Steps of Replica Symmetry Breaking
Chapter 10: Statistical Mechanics of Restricted Boltzmann Machine
-
10.1 Boltzmann Machine
-
10.2 Restricted Boltzmann Machine
-
10.3 Free Energy Calculation
-
10.4 Thermodynamic Quantities Related to Learning
-
10.5 Stability Analysis
-
10.6 Variational Mean-Field Theory for Training Binary RBMs
Chapter 11: Simplest Model of Unsupervised Learning with Binary
-
11.1 Model Setting
-
11.2 Derivation of sMP and AMP Equations
-
11.3 Replica Computation
-
11.4 Phase Transitions
-
11.5 Measuring the Temperature of Dataset
Chapter 12: Inherent-Symmetry Breaking in Unsupervised Learning
-
12.1 Model Setting
-
12.2 Phase Diagram
-
12.3 Hyper-Parameters Inference
Chapter 13: Mean-Field Theory of Ising Perceptron
-
13.1 Ising Perceptron model
-
13.2 Message-Passing-Based Learning
-
13.3 Replica Analysis
Chapter 14: Mean-Field Model of Multi-layered Perceptron
-
14.1 Random Active Path Model
-
14.2 Mean-Field Training Algorithms
-
14.3 Spike and Slab Model
Chapter 15: Mean-Field Theory of Dimension Reduction in Neural Networks
-
15.1 Mean-Field Model
-
15.2 Linear Dimensionality and Correlation Strength
-
15.3 Dimension Reduction with Correlated Synapses
Chapter 16: Chaos Theory of Random Recurrent Neural Networks
-
16.1 Spiking and Rate Models
-
16.2 Dynamical Mean-Field Theory
-
16.3 Lyapunov Exponent and Chaos
-
16.4 Excitation-Inhibition Balance Theory
-
16.5 Training Recurrent Neural Networks
Chapter 17: Statistical Mechanics of Random Matrices
-
17.1 Spectral Density
-
17.2 Replica Method and Semi-circle Law
-
17.3 Cavity Approach and Marchenko
-
17.4 Spectral Densities of Random Asymmetric Matrices
Chapter 18: Perspectives
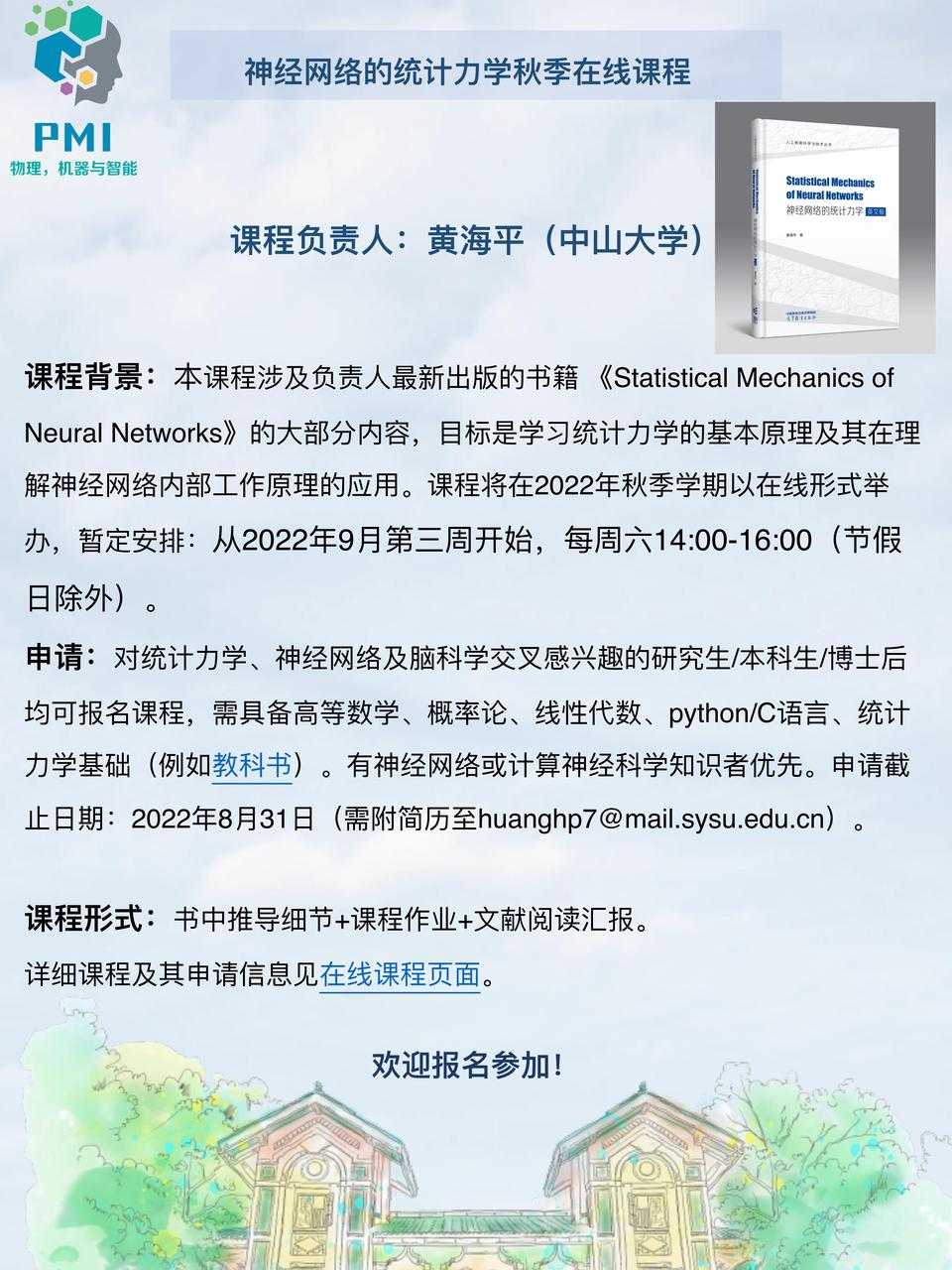