如何利用网络属性预测神经网络性能 | 周日直播·AI by Complexity读书会
导语

主题:网络属性决定神经网络模型性能
主题:网络属性决定神经网络模型性能
分享大纲
-
平均场近似与网络韧性
-
神经网络模型训练
-
神经网络到有向图
-
神经网络模型性能指标
-
模型筛选:实验结果
-
未来工作展望
-
Jiang, C., Huang, Z., Pedapati, T., Chen, P. Y., Sun, Y., & Gao, J. (2024). Network properties determine neural network performance. Nature Communications, 15(1), 1-9.
-
Gao, J., Barzel, B. & Barabási, A.-L. Universal resilience patterns in complex networks. Nature 530, 307–312 (2016).
-
Lillicrap, T. P., Santoro, A., Marris, L., Akerman, C. J. & Hinton, G. Backpropagation and the brain. Nat. Rev. Neurosci. 1–12 (2020).
-
Chandrashekaran, A. & Lane, I. R. Speeding up hyper-parameter optimization by extrapolation of learning curves using previous builds. In Joint European Conference on Machine Learning and Knowledge Discovery in Databases, 477–492 (Springer, 2017).
-
Nguyen, C., Hassner, T., Seeger, M. & Archambeau, C. LEEP: A new measure to evaluate transferability of learned representations. In International Conference on Machine Learning, 7294–7305 (PMLR, 2020).
-
Mellor, J., Turner, J., Storkey, A. & Crowley, E. J. Neural architecture search without training. In International Conference on Machine Learning, 7588–7598 (PMLR, 2021).
-
Dong, X., Liu, L., Musial, K. & Gabrys, B. NATS-Bench: Benchmarking nas algorithms for architecture topology and size. IEEE Transac. Pattern Anal. Machine Intelligence 7, 3634–3646 (2021).

直播信息
直播信息
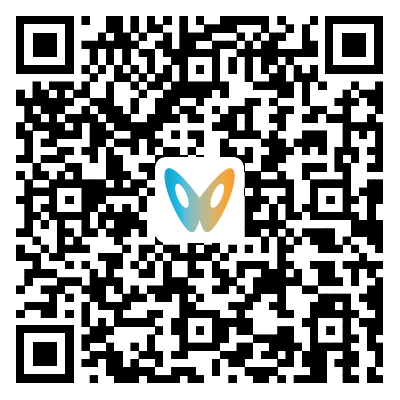
AI By Complexity读书会招募中
大模型、多模态、多智能体层出不穷,各种各样的神经网络变体在AI大舞台各显身手。复杂系统领域对于涌现、层级、鲁棒性、非线性、演化等问题的探索也在持续推进。而优秀的AI系统、创新性的神经网络,往往在一定程度上具备优秀复杂系统的特征。因此,发展中的复杂系统理论方法如何指导未来AI的设计,正在成为备受关注的问题。
集智俱乐部联合加利福尼亚大学圣迭戈分校助理教授尤亦庄、北京师范大学副教授刘宇、北京师范大学系统科学学院在读博士张章、牟牧云和在读硕士杨明哲、清华大学在读博士田洋共同发起「AI By Complexity」读书会,探究如何度量复杂系统的“好坏”?如何理解复杂系统的机制?这些理解是否可以启发我们设计更好的AI模型?在本质上帮助我们设计更好的AI系统。读书会于6月10日开始,每周一晚上20:00-22:00举办。欢迎从事相关领域研究、对AI+Complexity感兴趣的朋友们报名读书会交流!
往期分享:
课程链接:https://campus.swarma.org/course/1754
课程链接:https://campus.swarma.org/course/2328
点击“阅读原文”,报名读书会